The Transformative Impact of AI on the Future of Insurance: Strategic Insights for Industry Leaders
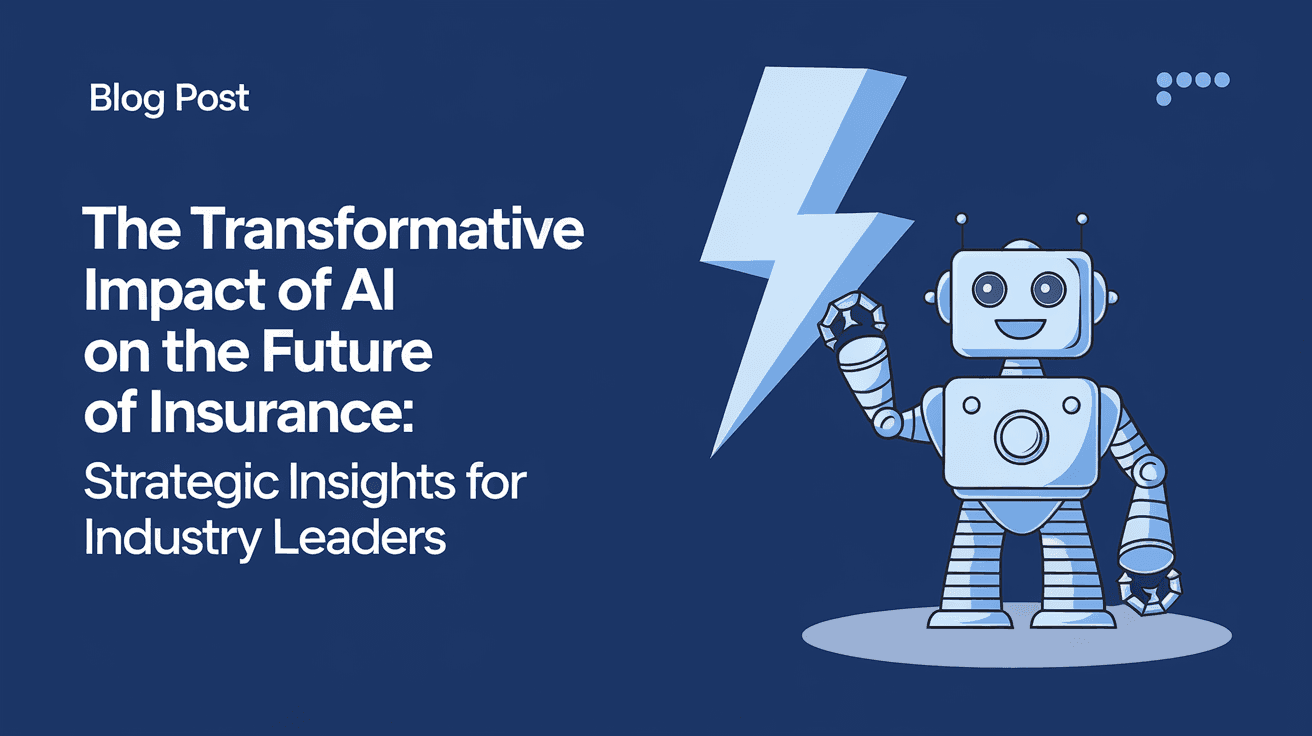
Table of Contents
- The Current State of AI in Insurance
- Core Areas Where AI is Reshaping Insurance
- Emerging AI-Driven Business Models
- Implementation Challenges and Solutions
- The Future Outlook: What's Next for AI in Insurance
- Strategic Recommendations for Insurance Executives
The Transformative Impact of AI on the Future of Insurance: Strategic Insights for Industry Leaders
The insurance industry stands at a technological crossroads. Artificial intelligence (AI) is fundamentally transforming how insurance companies assess risk, process claims, detect fraud, serve customers, and develop new products. For an industry built on data analysis and risk assessment, AI's capabilities to process vast datasets, recognize patterns, and enable predictive analytics represent both an unprecedented opportunity and a competitive necessity.
The integration of AI into insurance operations isn't merely about technological modernization—it's reshaping core business models and customer expectations. According to McKinsey, AI could potentially generate up to $1.1 trillion in annual value for the insurance industry. Yet many insurers struggle to move from pilot projects to scaled implementation, with only 25% of insurance companies having achieved significant AI deployment.
This article explores how AI is reshaping the insurance landscape, examines emerging business models, addresses implementation challenges, and provides strategic guidance for insurance executives navigating this transformation.
The Current State of AI in Insurance
Insurance has traditionally been a data-rich but insight-poor industry. While companies have collected vast amounts of information, the ability to transform this data into actionable intelligence has been limited by technology and processes. AI is changing this fundamental equation.
Currently, we're seeing varying levels of AI maturity across the insurance sector. Leaders in the space have implemented multiple AI use cases across their value chains, while many traditional insurers are still in experimental stages. A survey by Accenture found that 79% of insurance executives believe AI will revolutionize how they gain information from and interact with customers.
Key technologies driving this transformation include machine learning algorithms for risk assessment and pricing optimization, natural language processing (NLP) for claims management and customer service, computer vision for property assessment and damage evaluation, and predictive analytics for underwriting and fraud detection.
Early adopters are already seeing significant returns. For example, Lemonade, an insurtech pioneer, processes simple claims in seconds using its AI-powered claims bot, Jim. Similarly, traditional insurers like Ping An have reduced vehicle damage assessment time from days to minutes using AI-powered image recognition.
Core Areas Where AI is Reshaping Insurance
Underwriting and Risk Assessment
AI is fundamentally transforming the underwriting process—traditionally one of the most time-consuming and expertise-dependent areas of insurance. Machine learning algorithms can now analyze thousands of risk factors simultaneously, drawing insights from both structured and unstructured data sources.
This capability enables more accurate risk pricing based on individual behaviors rather than broad demographic categories, real-time underwriting decisions that can reduce application-to-policy time from weeks to minutes, identification of emerging risk patterns before they become apparent through traditional analytics, and integration of alternative data sources like social media, IoT devices, and satellite imagery.
For example, life insurers are increasingly using AI to assess risk from non-invasive sources like facial recognition technology that can identify smoking habits, biological age, and potential health issues without requiring medical examinations.
Claims Processing and Management
Claims processing represents one of the most promising areas for AI application. Traditional claims handling is labor-intensive, prone to errors, and often creates customer dissatisfaction due to lengthy processing times.
AI-driven claims solutions deliver automated first notice of loss (FNOL) intake through chatbots and virtual assistants, intelligent document processing that extracts information from submitted forms and images, automated damage assessment using computer vision, fraud detection algorithms that flag suspicious claims for investigation, and straight-through processing for simple, low-risk claims.
The results are compelling: insurers implementing AI in claims have reported 30% reduction in processing time and up to 50% increase in adjuster productivity.
Customer Experience and Personalization
Customer expectations in insurance are increasingly being shaped by experiences in other industries. AI enables insurers to deliver the personalized, responsive service that modern consumers demand.
Key applications include 24/7 customer service through intelligent chatbots and virtual assistants, personalized policy recommendations based on individual needs and risk profiles, proactive risk management advice using predictive analytics, simplified policy language through NLP-powered tools, and seamless omnichannel experiences across digital and human touchpoints.
Progressive insurers are using these capabilities to transform the customer relationship from transactional to advisory, positioning themselves as partners in risk management rather than just policy providers.
Fraud Detection and Prevention
Insurance fraud costs the industry billions annually. Traditional rule-based detection systems often generate false positives and miss sophisticated fraud schemes. AI-powered solutions are dramatically improving detection capabilities.
Advanced approaches include network analysis to identify connections between seemingly unrelated claims, anomaly detection that identifies patterns deviating from normal behavior, predictive models that score claims based on fraud likelihood, voice analysis during claims calls to detect potential deception, and social media mining to verify claim details.
These technologies not only improve detection rates but also enhance efficiency by allowing investigators to focus on high-probability cases while expediting legitimate claims.
Operational Efficiency
Beyond customer-facing functions, AI is driving significant operational improvements throughout insurance organizations.
Key operational applications include intelligent process automation for routine administrative tasks, advanced analytics for capacity planning and resource allocation, predictive maintenance for insured assets and infrastructure, natural language generation for automated reporting and documentation, and intelligent decision support systems for complex cases.
These efficiency gains translate directly to cost advantages. According to Accenture, AI could reduce operating costs by up to 40% while improving processing times and reducing error rates.
Emerging AI-Driven Business Models
AI isn't just improving existing operations—it's enabling entirely new business models that challenge traditional insurance paradigms.
Usage-Based Insurance
Telematics and IoT devices combined with AI analytics are powering the growth of usage-based insurance (UBI), particularly in auto insurance. Rather than static risk assessments, UBI uses real-time driving data to continuously adjust premiums based on actual behavior.
This model delivers more accurate risk-based pricing, incentives for safer behavior, reduced premiums for low-risk customers, and enhanced customer engagement through feedback loops. Progressive's Snapshot program and Allstate's Drivewise are leading examples of this approach, with AI algorithms processing driving data to determine personalized pricing.
On-Demand Coverage
AI's ability to assess risk in real-time supports the growing market for on-demand insurance—coverage that can be turned on and off as needed through a mobile app.
This model appeals particularly to younger consumers and the sharing economy, offering coverage only when needed (reducing overall cost), instant activation and deactivation, simplified purchasing process, and customization for specific activities or timeframes. Companies like Trov pioneered this approach for item-specific insurance, while Cuvva offers hourly car insurance in the UK.
Parametric Insurance
AI-powered parametric insurance automatically pays out when specific, measurable parameters are met, eliminating the claims process entirely.
This model works particularly well for natural disaster coverage, crop insurance, travel delay protection, and business interruption insurance. For example, FloodFlash uses IoT sensors to measure flood levels and automatically triggers payments when water reaches predetermined depths. This approach delivers immediate liquidity after loss events without requiring claims adjustment.
Micro-Insurance Innovations
AI's ability to reduce operational costs makes previously unprofitable micro-insurance models viable for underserved markets.
These solutions provide affordable coverage for specific risks, streamlined distribution through digital channels, automated administration to reduce costs, and risk assessment for populations with limited data history. BIMA provides microinsurance in emerging markets through mobile technology, using AI to assess risk and manage policies for customers with no previous insurance history.
Implementation Challenges and Solutions
Despite the compelling benefits, insurance companies face significant challenges in implementing AI at scale.
Data Quality and Integration Issues
Insurance data is often fragmented across legacy systems, inconsistent in format, and of varying quality. AI models are only as good as the data they're trained on.
Solutions include implementing enterprise data lakes to centralize information, data cleansing and enrichment pipelines, synthetic data generation for underrepresented scenarios, APIs and middleware for legacy system integration, and data governance frameworks to ensure ongoing quality. Leading insurers are establishing dedicated data offices responsible for treating data as a strategic asset rather than a byproduct of operations.
Talent and Skill Gaps
The insurance industry competes with tech giants and startups for scarce AI talent. Building internal capabilities requires both recruitment and retraining strategies.
Effective approaches include creating specialized AI centers of excellence, partnerships with universities and research institutions, acquisition of insurtech companies with established AI capabilities, retraining actuaries and data analysts in machine learning techniques, and collaboration with AI solution providers for knowledge transfer. Organizations like Business+AI facilitate these connections through workshops and masterclasses designed to build practical AI implementation skills.
Regulatory and Compliance Considerations
AI introduces new regulatory challenges around explainability, bias, and data privacy. Insurance is already heavily regulated, and AI deployments must navigate this complex landscape.
Key considerations include explainable AI requirements for underwriting decisions, bias testing and mitigation in algorithmic systems, GDPR and other data privacy compliance, emerging AI-specific regulations, and model risk management frameworks. Leading insurers are proactively engaging with regulators and participating in industry standards development to shape the regulatory environment.
Change Management Hurdles
Perhaps the most significant challenge is organizational change management. AI implementation requires new workflows, skills, and mindsets throughout the organization.
Successful transformations include executive-level AI champions with transformation authority, clear communication of AI's strategic importance, early wins to demonstrate value and build momentum, cross-functional implementation teams, and incentive alignment for AI adoption. Insurers that approach AI as a strategic transformation rather than a series of technology projects are seeing higher success rates and better returns on investment.
The Future Outlook: What's Next for AI in Insurance
Looking ahead, several trends will shape AI's continued impact on insurance:
Autonomous Insurance Operations
The future of insurance will include fully autonomous processes that require human intervention only for exceptions and strategic decisions. This includes end-to-end automated underwriting for standard risks, self-optimizing pricing models that adjust to market conditions, fully automated claims processing for up to 80% of cases, and AI-driven product development based on emerging risk patterns.
Munich Re is already moving in this direction with automated underwriting for certain life insurance products, dramatically reducing processing time and costs.
Ecosystem Integration
AI will enable deeper integration between insurers and external ecosystems, creating new value propositions: connected health ecosystems that combine insurance with wellness monitoring, smart home systems with integrated property insurance, embedded insurance in automotive and transportation platforms, and risk management services integrated with commercial coverage.
Ping An's Good Doctor platform illustrates this trend, combining health insurance with AI-powered medical consultations and wellness services.
Quantum Computing and Advanced AI
Longer-term developments in quantum computing will unlock new capabilities for risk modeling and simulation: real-time processing of vastly more complex risk models, quantum machine learning for previously intractable problems, simulation capabilities that predict emerging risks before historical data exists, and personalized risk modeling at an unprecedented scale.
Leading insurers are already partnering with quantum computing researchers to prepare for this next wave of innovation.
Human-AI Collaboration Models
The most successful insurers will develop effective models for human-AI collaboration: AI handling routine decisions while humans focus on complex cases, augmented intelligence tools that enhance human capabilities, ethical oversight frameworks for algorithmic decisions, and new roles focusing on AI training, monitoring, and improvement.
This collaborative approach will maintain the human judgment and empathy essential in insurance while leveraging AI's analytical power.
Strategic Recommendations for Insurance Executives
For insurance leaders navigating this transformation, several strategic priorities emerge:
Develop an AI Roadmap with Business Outcomes
Successful AI implementation starts with clear business objectives rather than technology experiments: identify high-value use cases based on business impact, establish measurable KPIs for each initiative, create a sequenced implementation plan with quick wins and long-term goals, and align AI investments with overall strategic priorities.
Business+AI consulting services can help organizations develop roadmaps tailored to their specific business needs and maturity level.
Build the Right Data Foundation
Without quality data, AI initiatives will struggle to deliver value: audit existing data assets and identify gaps, implement data governance programs, invest in data integration infrastructure, create feedback loops that continuously improve data quality, and develop synthetic data capabilities for scenarios with limited historical data.
This foundation should be built with specific AI use cases in mind to ensure relevance and focus.
Focus on Change Management and Talent Development
The human element remains critical to AI success: develop AI literacy programs for all staff, create centers of excellence to concentrate technical expertise, establish clear roles and responsibilities for AI systems, redesign processes and workflows to incorporate AI capabilities, and implement agile methodologies for AI development and deployment.
Organizations seeking to accelerate this transformation can leverage Business+AI workshops and masterclasses to build internal capabilities.
Create an Ethical AI Framework
Responsible AI deployment requires proactive ethical governance: develop principles for fair and unbiased algorithmic decisions, create transparency in how AI influences customer outcomes, establish oversight mechanisms for AI systems, regularly audit systems for unintended consequences, and engage with regulators and industry standards organizations.
This ethical foundation builds customer trust while reducing regulatory and reputational risks.
Form Strategic Partnerships
Few insurers can develop all necessary AI capabilities internally: partner with AI technology providers for specific capabilities, collaborate with insurtech startups for innovation, engage with industry forums like the Business+AI Forum to share best practices, create academic partnerships for research and talent development, and consider consortium approaches for industry-wide challenges.
These partnerships can accelerate implementation while providing access to specialized expertise.
Conclusion
AI is not simply another technology innovation for insurance—it represents a fundamental transformation of the industry's core processes, business models, and value proposition. The most successful insurers will be those who approach AI strategically, focusing on business outcomes rather than technology for its own sake.
The journey requires addressing significant challenges in data quality, talent development, regulatory compliance, and organizational change. However, those who navigate these challenges successfully will create substantial competitive advantages through enhanced customer experiences, operational efficiency, and innovative products.
For insurance executives, the time for AI experimentation has passed. The industry has moved into an implementation phase where scaled deployment is creating measurable value. Those who delay risk finding themselves at a significant competitive disadvantage as AI-powered insurers set new standards for cost, speed, and customer experience.
The future of insurance will be defined by organizations that can effectively combine human expertise with AI capabilities, creating systems that are more accurate, efficient, and responsive than either humans or machines could achieve independently.
Ready to Transform Your Business with AI?
Join the Business+AI ecosystem to accelerate your organization's AI journey. Our membership program connects you with AI experts, solution providers, and fellow executives navigating similar challenges. Through hands-on workshops, masterclasses, and our annual forum, we help turn AI potential into tangible business results.