The Transformative Impact of AI in Banking: From Automation to Intelligence
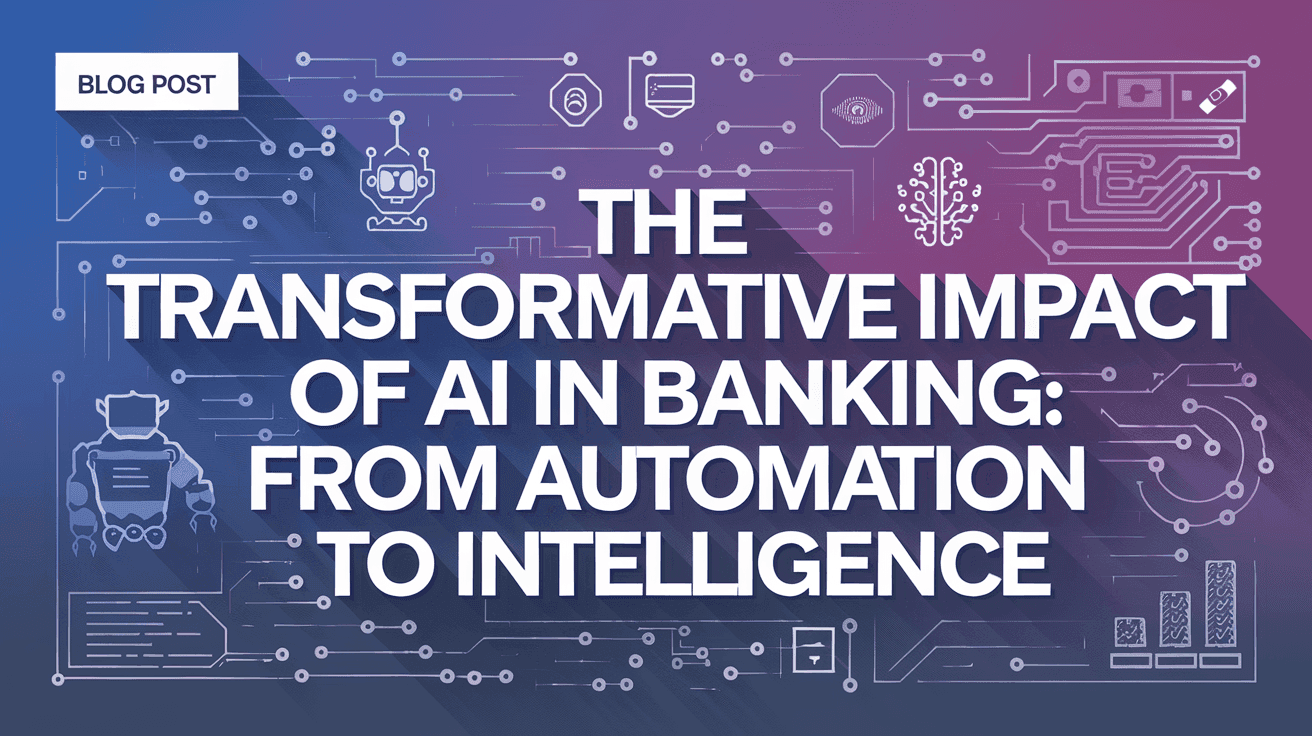
Table of Contents
- The AI Banking Revolution: Key Applications and Use Cases
- Generative AI: The New Frontier in Banking
- Building an Effective AI Operating Model for Banks
- Implementation Roadmap: From Strategy to Execution
- Navigating the Regulatory Landscape
- The Future of AI in Banking
The Transformative Impact of AI in Banking: From Automation to Intelligence
Artificial Intelligence (AI) has evolved from an experimental technology to a strategic imperative in the banking sector. What began as basic automation tools has transformed into sophisticated systems capable of learning, predicting, and even generating content that can revolutionize how financial institutions operate and serve their customers.
Today, banks worldwide are implementing AI solutions across their value chains - from customer-facing interactions to back-office operations, risk management, and strategic decision-making. According to McKinsey, AI technologies could potentially deliver up to $1 trillion in additional value annually for the global banking industry. Despite this enormous potential, many institutions struggle to move beyond pilot projects and proof-of-concepts to implement AI at scale.
In this comprehensive guide, we'll explore the diverse applications of AI in banking, examine the transformative potential of generative AI, provide frameworks for building effective AI operating models, and offer practical guidance for implementation. Whether you're a banking executive, technology leader, or consultant supporting the financial services sector, this article will help you navigate the complex landscape of AI in banking and turn technological possibilities into tangible business outcomes.
The AI Banking Revolution: Key Applications and Use Cases
Customer Experience and Service
AI has dramatically transformed how banks interact with their customers, creating more personalized, efficient, and engaging experiences.
AI-powered chatbots and virtual assistants have evolved beyond simple FAQ responders to become sophisticated service agents. Leading banks in Singapore and across Asia have deployed virtual assistants that can help customers with complex transactions, resolve disputes, and even provide financial advice. These AI-powered assistants operate 24/7, reducing wait times and handling up to 80% of routine customer inquiries without human intervention.
Personalized financial recommendations represent another powerful application of AI in banking. By analyzing transaction histories, spending patterns, and financial goals, AI systems can offer tailored savings strategies, investment opportunities, and product recommendations. DBS Bank, for instance, uses its AI-powered financial GPS to provide customers with hyper-personalized insights and recommendations, resulting in a 33% increase in product adoption rates.
Enhanced mobile banking experiences leverage AI to create intuitive, predictive interfaces that anticipate customer needs. Features like predictive text, voice banking, and smart notifications make mobile banking more accessible and user-friendly. According to Accenture, banks that have implemented AI in their mobile banking apps have seen customer engagement increase by up to 40%.
Risk Management and Compliance
AI is revolutionizing how banks manage risk and maintain regulatory compliance, allowing for more accurate, efficient, and proactive approaches.
Fraud detection and prevention systems powered by AI can analyze thousands of transactions per second, identifying suspicious patterns that human analysts might miss. These systems continuously learn from new data, adapting to evolving fraud tactics in real-time. Major banks have reported up to 60% reduction in false positives and 50% faster fraud detection after implementing AI-based solutions.
Credit scoring and underwriting has been transformed by AI's ability to analyze alternative data sources beyond traditional credit reports. Advanced machine learning models can evaluate thousands of variables - from spending patterns to social media activity - to assess creditworthiness. This enables banks to make more accurate lending decisions and extend credit to previously underserved segments, including thin-file customers with limited credit history.
AML and KYC automation addresses one of banking's most resource-intensive compliance requirements. AI-powered systems can verify customer identities, monitor transactions for suspicious activities, and generate regulatory reports with minimal human intervention. According to KPMG, financial institutions can reduce AML compliance costs by up to 40% through effective AI implementation.
Operational Efficiency
Banks are leveraging AI to streamline operations, reduce costs, and improve productivity across their organizations.
Process automation in back-office operations goes beyond traditional RPA (Robotic Process Automation) with intelligent systems that can handle exceptions and make decisions based on context. AI-powered automation in areas like account reconciliation, payment processing, and reporting has enabled banks to reduce processing times by up to 90% while improving accuracy.
Document processing and data extraction solutions use computer vision and natural language processing to automate the handling of unstructured documents. From loan applications to compliance documentation, AI systems can extract relevant information, verify completeness, and route documents appropriately. Banks implementing these solutions have reduced document processing time from days to minutes.
Workforce augmentation and productivity tools help bank employees work more efficiently by automating routine tasks and providing decision support. AI assistants can help relationship managers prepare for client meetings, suggest next-best actions, and handle administrative tasks, allowing them to focus on higher-value activities. According to Deloitte, AI-powered workforce augmentation can improve employee productivity by up to 35% in financial services.
Financial Intelligence and Decision-Making
AI is enhancing banks' ability to derive insights from data and make strategic decisions with greater confidence and precision.
Advanced analytics for market insights combine traditional data analysis with AI to identify market trends, competitive movements, and customer preferences. These insights enable banks to develop more targeted products, optimize pricing strategies, and identify new market opportunities. Leading banks are using AI-powered analytics to gain a competitive edge in rapidly evolving markets.
Investment management and robo-advisors leverage AI to democratize wealth management services. Algorithmic investment platforms can create diversified portfolios tailored to individual risk profiles and financial goals, making investment advice accessible to a broader customer base. Major banks have launched hybrid models combining AI-driven recommendations with human advisors for higher-value clients.
Predictive analytics for business strategy help banks forecast market changes, anticipate customer needs, and optimize resource allocation. AI models can simulate various scenarios, helping leaders make more informed strategic decisions. From branch network optimization to product development prioritization, predictive analytics are becoming essential tools for strategic planning in banking.
Generative AI: The New Frontier in Banking
Generative AI represents the latest evolution in artificial intelligence, with transformative potential for the banking sector. Unlike traditional AI that analyzes and predicts based on existing data, generative AI can create new content, from text and images to code and conversational responses.
Defining generative AI and its unique capabilities is essential to understanding its potential impact. Generative AI systems, particularly large language models (LLMs) like GPT-4, can understand context, generate human-like text, summarize complex documents, and even write code. This represents a fundamental shift from rule-based automation to creative, adaptive intelligence that can handle nuanced tasks requiring judgment and communication.
Key applications of generative AI in banking are rapidly emerging across functions:
-
Enhanced customer interactions: Banks are using generative AI to power more sophisticated conversational interfaces that can understand and respond to complex customer queries in natural language, handle multiple topics in a single conversation, and maintain context over extended interactions.
-
Content creation and personalization: From crafting personalized financial advice to generating customized investment reports, generative AI is helping banks scale their content creation while maintaining relevance for individual customers.
-
Code generation and development: IT teams are leveraging generative AI to accelerate application development, automate testing, and write documentation, significantly reducing development time and costs.
-
Document analysis and processing: Generative AI can extract key information from complex financial documents, summarize lengthy reports, and generate concise briefings, saving significant time for analysts and decision-makers.
The economic potential and projected impact of generative AI in banking is substantial. McKinsey estimates that generative AI could add between $200 billion and $340 billion in value annually to the global banking sector, representing 2.8 to 4.7 percent of total industry revenues. This value creation will come primarily through productivity improvements, enhanced customer experiences, and new product innovations.
Implementation challenges specific to generative AI include:
-
Data privacy and security concerns: Generative AI requires access to large datasets, raising questions about data governance and protection.
-
Accuracy and hallucination issues: Current generative AI models can sometimes produce plausible-sounding but factually incorrect information, requiring careful validation mechanisms.
-
Regulatory uncertainty: The rapidly evolving capabilities of generative AI are outpacing regulatory frameworks, creating compliance challenges for banks.
-
Integration with existing systems: Connecting generative AI capabilities with legacy banking infrastructure presents technical challenges.
Despite these challenges, generative AI is poised to become a competitive differentiator for banks that can effectively harness its capabilities while managing the associated risks.
Building an Effective AI Operating Model for Banks
Implementing AI at scale requires more than just technology; it demands a carefully designed operating model that aligns with your organization's structure, culture, and strategic objectives. Based on research and experience with leading financial institutions, four primary operating model approaches have emerged:
Four Key Operating Model Approaches
Highly centralized model: In this approach, a central AI team handles everything from strategy to implementation. This team operates independently, with dedicated resources and authority to drive AI initiatives across the organization.
Benefits: Faster capability building, consistent standards, efficient resource allocation, and streamlined decision-making.
Challenges: Potential disconnect from business units, risk of prioritizing technically interesting projects over business-critical needs, and possible resistance from business units.
Centrally led, business-unit executed model: Here, a central team sets strategy and standards while working closely with business units for implementation. The central team provides expertise, tools, and oversight, while business units contribute domain knowledge and handle day-to-day execution.
Benefits: Better alignment with business priorities, stronger buy-in from business units, and balanced technical and business perspectives.
Challenges: Slower execution due to coordination requirements, potential resource constraints in business units, and governance complexity.
Business-unit led, centrally supported model: In this approach, business units drive AI initiatives based on their specific needs, while a central team provides standards, tools, and technical support. Business units have primary decision-making authority for their AI projects.
Benefits: Strong alignment with immediate business needs, rapid response to market opportunities, and clear ownership within business units.
Challenges: Inconsistent implementation across the organization, potential duplication of efforts, and difficulty scaling successful use cases enterprise-wide.
Highly decentralized model: Each business unit independently develops and implements its own AI capabilities with minimal central coordination. Units build their own AI teams and establish their own processes and standards.
Benefits: Maximum responsiveness to business unit needs, clear accountability, and specialized solutions tailored to specific contexts.
Challenges: Inefficient resource allocation, inconsistent standards, significant duplication of effort, and difficulty sharing lessons learned across the organization.
Selecting the Right Model for Your Organization
Research from McKinsey and other sources indicates that in the current stage of AI maturity, more centralized approaches tend to yield better results. Approximately 70% of banks with highly centralized AI operating models have successfully deployed AI use cases into production, compared to only 30% of those with fully decentralized approaches.
However, the ideal model depends on several organizational factors:
-
AI maturity: Organizations in early stages of AI adoption often benefit from more centralized approaches to build foundational capabilities and standards.
-
Organizational structure: The existing structure of your bank (centralized vs. federated) will influence which AI operating model will face less resistance.
-
Resource availability: The availability of AI talent and technology infrastructure may dictate whether resources should be pooled centrally or distributed.
-
Strategic priorities: If AI is viewed as a transformational force requiring rapid, coordinated change, a more centralized model may be appropriate.
-
Regulatory environment: Banks operating in highly regulated environments may benefit from centralized oversight of AI risk and compliance.
Many successful organizations have adopted hybrid approaches, centralizing certain functions (like risk management and technical standards) while distributing others (like use case identification and business integration).
Essential Components of a Successful AI Operating Model
Regardless of the specific model chosen, effective AI operating models in banking share several critical components:
-
Clear governance structures: Defined roles, responsibilities, and decision rights for AI initiatives, including executive sponsorship and oversight.
-
Standardized processes: Consistent methodologies for use case identification, prioritization, development, and deployment across the organization.
-
Technology infrastructure: Shared platforms, tools, and environments that enable efficient development and deployment of AI solutions.
-
Data governance: Established protocols for data access, quality, privacy, and security to support AI development while managing risk.
-
Talent management: Strategies for attracting, developing, and retaining AI specialists, as well as upskilling the broader workforce.
-
Risk management framework: Comprehensive approaches to identify, assess, and mitigate AI-specific risks, including bias, explainability, and regulatory compliance.
-
Value tracking: Mechanisms to measure and monitor the business impact of AI initiatives, ensuring accountability and continued support.
As AI technologies mature and organizations gain experience, operating models typically evolve from more centralized to more federated approaches, allowing for greater innovation and customization while maintaining essential standards and oversight.
Implementation Roadmap: From Strategy to Execution
Successful AI implementation in banking requires a structured approach that addresses technical, organizational, and human factors. Here's a practical roadmap for turning AI potential into tangible business outcomes:
Assessing AI Readiness and Opportunity Areas
Begin by evaluating your organization's current capabilities and identifying the most promising applications of AI:
-
Capability assessment: Evaluate your existing technology infrastructure, data assets, talent, and organizational processes to identify strengths and gaps.
-
Opportunity identification: Conduct a systematic review of business processes and customer journeys to identify high-value AI use cases. Prioritize opportunities based on potential impact, feasibility, and strategic alignment.
-
Benchmark analysis: Compare your AI capabilities and adoption to industry peers to identify competitive gaps and opportunities for differentiation.
Leading banks typically identify 20-30 potential AI use cases during this phase, then narrow down to 5-10 high-priority initiatives for initial implementation.
Building the Business Case and Securing Resources
Translate identified opportunities into compelling business cases that secure necessary support:
-
Value quantification: Estimate both tangible benefits (cost savings, revenue growth) and intangible benefits (improved customer experience, reduced risk) for each AI initiative.
-
Cost modeling: Develop comprehensive cost estimates covering technology, talent, change management, and ongoing operations.
-
Risk assessment: Identify potential implementation risks and develop mitigation strategies to address them.
-
Funding strategy: Determine appropriate funding mechanisms, whether through central innovation budgets, business unit allocations, or separate transformation funding.
Successful business cases typically demonstrate ROI within 12-18 months for initial use cases, with plans for scaling and expanding impact over 3-5 years.
Technology Infrastructure Considerations
Establish the technical foundation to support AI development and deployment at scale:
-
Platform strategy: Decide whether to build custom AI platforms, leverage cloud-based AI services, or adopt a hybrid approach based on your specific requirements.
-
Tool selection: Evaluate and select appropriate tools for data processing, model development, deployment, monitoring, and governance.
-
Integration architecture: Design approaches for integrating AI capabilities with existing core banking systems, customer channels, and third-party services.
-
Technical standards: Establish standards for model development, testing, deployment, and documentation to ensure consistency and quality.
Many banks are adopting modular, cloud-based architectures that provide flexibility, scalability, and access to cutting-edge AI capabilities without requiring complete system overhauls.
Data Management and Governance Requirements
Address data challenges that often represent the biggest hurdles to AI success:
-
Data strategy: Develop a comprehensive approach to data collection, storage, access, and usage that supports AI initiatives while ensuring compliance.
-
Quality improvement: Implement processes to enhance data quality, completeness, and consistency across systems.
-
Governance framework: Establish policies and procedures for data access, usage, privacy, and security specific to AI applications.
-
Ethical guidelines: Develop principles and review processes to ensure AI systems use data in ways that are ethical, fair, and align with organizational values.
Banks that excel in AI implementation typically centralize data governance while enabling appropriate access for AI teams, striking a balance between control and innovation.
Talent Acquisition and Development Strategies
Address the critical human element of AI implementation:
-
Skills assessment: Identify required AI roles and skills, from data scientists and ML engineers to AI ethicists and translators who bridge technical and business domains.
-
Talent strategy: Develop a multi-pronged approach to building AI capabilities through hiring, upskilling, partnerships, and outsourcing.
-
Organizational design: Structure teams to support collaboration between AI specialists and business domain experts.
-
Learning programs: Create continuous learning opportunities to keep teams current with rapidly evolving AI technologies and applications.
Successful banks are creating hybrid teams that combine deep technical expertise with banking domain knowledge, often organizing them into agile, cross-functional squads focused on specific use cases or domains.
Managing Change and Driving Adoption
Ensure that AI solutions deliver their intended impact through effective change management:
-
Stakeholder engagement: Identify and engage key stakeholders early to build understanding, address concerns, and secure support.
-
Communication strategy: Develop clear messaging about the purpose, benefits, and impact of AI initiatives for different audiences.
-
Training and support: Prepare employees to work with new AI tools and adapt to changing roles and processes.
-
Success measurement: Establish clear metrics and feedback mechanisms to track adoption and impact, enabling continuous improvement.
Change management is particularly critical for AI initiatives that affect customer-facing roles or significantly alter established work processes. Leading banks dedicate 15-20% of project resources to change management activities to ensure successful adoption.
Navigating the Regulatory Landscape
The regulatory environment for AI in banking is evolving rapidly, creating both challenges and opportunities for financial institutions.
Current Regulatory Frameworks for AI in Banking
Regulatory approaches to AI in banking vary by region but generally focus on several key areas:
-
Consumer protection: Ensuring AI-based decisions are fair, transparent, and do not discriminate against protected groups.
-
Explainability: Requiring that banks can explain how AI models make decisions, particularly for credit, pricing, and risk assessments.
-
Data privacy: Governing how customer data can be collected, used, and shared for AI development and deployment.
-
System resilience: Ensuring AI systems are reliable, secure, and can be properly overseen by humans when necessary.
In Singapore, the Monetary Authority of Singapore (MAS) has been at the forefront of AI regulation in banking, publishing guidelines on Fairness, Ethics, Accountability and Transparency (FEAT) that have become influential globally. The European Union's AI Act and the U.S. approach through existing regulatory frameworks represent other significant developments.
Risk Management and Compliance Considerations
Banks must develop comprehensive approaches to managing AI-specific risks:
-
Model risk management: Extending traditional model governance frameworks to address unique characteristics of AI systems, including complexity, opacity, and adaptability.
-
Bias detection and mitigation: Implementing processes to identify and address potential biases in AI systems, including regular testing and monitoring.
-
Third-party risk management: Establishing controls for AI capabilities sourced from vendors or developed using third-party tools and platforms.
-
Documentation requirements: Creating comprehensive documentation of AI development, testing, validation, and ongoing monitoring to satisfy regulatory requirements.
Leading banks are establishing dedicated AI governance committees that bring together technology, risk, legal, and business perspectives to provide oversight of AI initiatives.
Ethical AI Implementation and Governance
Beyond regulatory compliance, banks must address broader ethical considerations:
-
AI ethics frameworks: Developing principles and guidelines for responsible AI development and use that reflect organizational values.
-
Ethics review processes: Establishing mechanisms to assess proposed AI applications against ethical standards before development begins.
-
Ongoing monitoring: Implementing continuous monitoring of deployed AI systems to detect potential ethical issues or unintended consequences.
-
Stakeholder engagement: Engaging with customers, employees, regulators, and other stakeholders to understand and address concerns about AI use.
Banks that proactively address ethical considerations often find they are better positioned to navigate regulatory requirements and build trust with customers and other stakeholders.
Regional Variations in AI Banking Regulation
Banks operating globally must navigate varying regulatory approaches:
-
Asia-Pacific: Singapore, Hong Kong, and Australia have taken proactive approaches to AI governance, developing specific guidelines while encouraging innovation.
-
European Union: The EU's approach emphasizes consumer protection and data privacy, with strict requirements for explainability and fairness in high-risk applications.
-
North America: The U.S. has focused on applying existing regulatory frameworks to AI applications, with increasing attention to fairness and transparency.
-
Emerging markets: Many developing economies are working to balance innovation and consumer protection, often adapting frameworks from more established markets.
Global banks are developing modular approaches to AI governance that allow for adaptation to regional requirements while maintaining consistent core principles and standards.
The Future of AI in Banking
As AI technologies continue to evolve and mature, the banking landscape will undergo further transformation. Forward-thinking institutions are already preparing for the next wave of innovation.
Emerging Trends and Technologies
Several developments are likely to shape the future of AI in banking:
-
Multimodal AI: Systems that can process and generate multiple types of content (text, images, voice) will enable more natural and comprehensive customer interactions.
-
Edge AI: Moving AI processing closer to where data is generated will enable faster, more private, and more resilient applications, particularly for mobile banking.
-
Quantum AI: The convergence of quantum computing and AI promises to solve previously intractable problems, particularly in risk modeling and optimization.
-
AI-human collaboration models: More sophisticated approaches to combining human expertise with AI capabilities will emerge, creating new roles and workflows.
-
Federated learning: Privacy-preserving techniques that allow AI models to learn from data without centralizing it will address key regulatory and security concerns.
As these technologies mature, they will enable new applications that are currently not feasible due to technical, regulatory, or economic constraints.
Long-term Impact on Banking Business Models
AI will fundamentally reshape banking business models in several ways:
-
Extreme personalization: Banks will move beyond segmentation to true 1:1 personalization of products, services, pricing, and interactions, enabled by AI's ability to process vast amounts of data and make real-time decisions.
-
Embedded banking: AI will facilitate the seamless integration of banking services into non-financial platforms and experiences, making banking more contextual and invisible.
-
Autonomous finance: AI systems will increasingly manage routine financial decisions and transactions on behalf of customers, from bill payments to investment rebalancing.
-
New value propositions: Banks will leverage AI to offer entirely new services, such as predictive financial planning, personalized risk management, and data-driven business advisory services.
-
Operating model transformation: The boundary between technology and banking functions will continue to blur, with AI capabilities embedded throughout the organization rather than siloed in specialized departments.
These shifts will require banks to reevaluate their core value propositions, competitive positioning, and organizational capabilities.
Preparing for the Next Wave of AI Innovation
To position themselves for future success, banks should take several proactive steps:
-
Establish AI innovation radar: Develop systematic processes to monitor emerging AI technologies, use cases, and competitive developments.
-
Build strategic partnerships: Form relationships with AI startups, academic institutions, and technology providers to access emerging capabilities and talent.
-
Create experimentation frameworks: Establish structured approaches to testing new AI technologies and applications with appropriate governance and risk management.
-
Develop scenario plans: Prepare for alternative futures by developing scenarios that consider different trajectories of AI development and adoption.
-
Cultivate adaptability: Build organizational capabilities for rapid adaptation, including modular technology architecture, flexible team structures, and agile governance processes.
Banks that combine a strong foundation in current AI capabilities with forward-looking preparation will be best positioned to thrive as the technology continues to evolve.
Conclusion
AI is transforming banking from a technology experiment to a business imperative. From enhancing customer experiences to revolutionizing risk management, improving operational efficiency, and enabling more sophisticated decision-making, AI applications are creating value across the banking value chain.
The emergence of generative AI represents the next frontier, with potential to deliver hundreds of billions in value to the global banking sector. However, realizing this potential requires more than technology investment—it demands thoughtful approaches to operating models, implementation, regulatory compliance, and future planning.
As we've explored throughout this article, successful AI implementation in banking requires:
- A clear understanding of high-value use cases across the organization
- An appropriate operating model that balances centralization and business unit ownership
- A structured implementation approach addressing technology, data, talent, and change management
- Comprehensive governance and risk management frameworks
- Forward-looking strategies to adapt to evolving technologies and business models
Banks that excel in these dimensions will not only capture immediate efficiency gains but also position themselves for sustainable competitive advantage in an increasingly AI-powered financial services landscape.
The journey to becoming an AI-enabled bank is challenging but essential for long-term success. By taking a strategic, comprehensive approach—balancing innovation with risk management, technical capabilities with business alignment, and current needs with future possibilities—banks can turn AI from a buzzword into a powerful driver of business value.
Ready to accelerate your AI journey in banking? Business+AI offers a comprehensive ecosystem to help financial institutions turn AI potential into tangible business outcomes.
Explore our workshops and masterclasses for hands-on learning and skill development in AI implementation. Connect with industry peers and solution providers at our flagship Business+AI Forum to share insights and best practices.
For organizations seeking more personalized guidance, our consulting services provide expert support for AI strategy development, use case identification, operating model design, and implementation planning.
Join the Business+AI membership program today to access our full range of resources, events, and expertise, and position your organization at the forefront of AI-powered banking innovation.