The Transformative Impact of AI and Robotics on Healthcare: From Innovation to Implementation
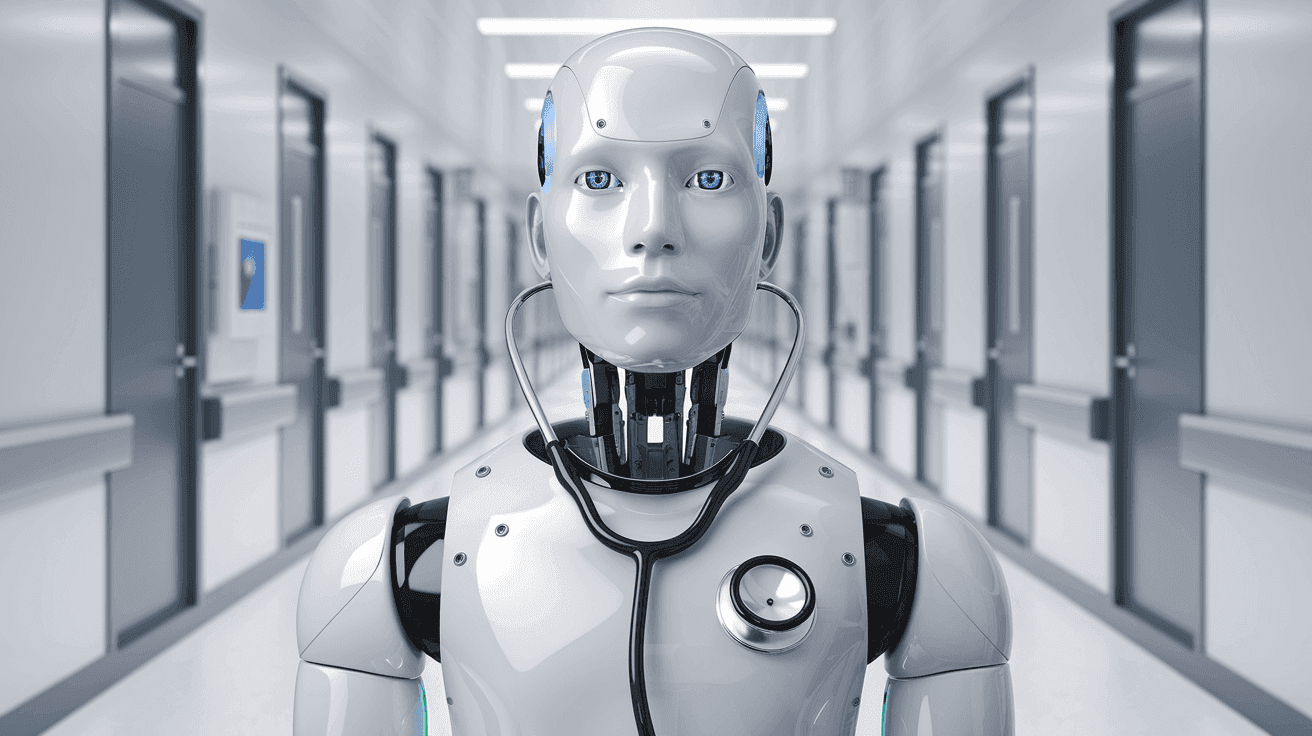
Table of Contents
- The Business Case for AI and Robotics in Healthcare
- Eight Transformative Applications in Healthcare
- Implementation Challenges and Solutions
- Regulatory and Ethical Considerations
- The Path Forward
The Transformative Impact of AI and Robotics on Healthcare: From Innovation to Implementation
In Singapore's Changi General Hospital, an AI system analyzes thousands of chest X-rays daily, flagging potential abnormalities for radiologists and reducing diagnosis time by 80%. Meanwhile, in operating rooms across Southeast Asia, surgical robots assist doctors in performing minimally invasive procedures with unprecedented precision, reducing recovery times and improving outcomes.
These aren't futuristic scenarios—they're happening right now. Artificial intelligence and robotics are fundamentally transforming healthcare delivery worldwide, creating a projected market value of $187.95 billion by 2030.
For healthcare executives and business leaders, this technological revolution represents both a challenge and an opportunity. How do you separate hype from reality? Which technologies deserve investment? How can implementation be managed effectively while navigating complex regulatory environments?
This article examines how AI and robotics are reshaping healthcare business models, clinical outcomes, and patient experiences. We'll explore practical applications, implementation strategies, and the critical considerations for leaders looking to harness these technologies for tangible business results in the healthcare sector.
The Business Case for AI and Robotics in Healthcare
The integration of AI and robotics into healthcare isn't merely a technological evolution—it represents a fundamental business transformation. Healthcare organizations implementing AI solutions report an average cost reduction of 25.7% while simultaneously improving patient outcomes.
In Singapore and across Southeast Asia, several factors make the business case particularly compelling:
- Aging populations creating unprecedented demand for healthcare services
- Shortage of healthcare professionals necessitating efficiency-enhancing technologies
- Growing middle class with increasing expectations for healthcare quality
- Government initiatives supporting healthcare digitalization
A Boston Consulting Group study found that healthcare providers implementing AI solutions for operational workflows achieved ROI within 14 months on average. For diagnostic applications, the timeline extended to 20 months, but with significantly higher long-term returns.
The business imperative is clear: healthcare organizations that strategically implement AI and robotics gain competitive advantages through improved efficiency, enhanced clinical outcomes, and better patient experiences—ultimately translating to stronger financial performance and market positioning.
Eight Transformative Applications in Healthcare
Preventative Care and Wellness
AI's most valuable contribution may be keeping patients healthy and out of hospitals altogether. Predictive analytics and machine learning algorithms now identify high-risk individuals before symptoms appear, enabling targeted interventions that prevent disease progression.
Singapore's Health Promotion Board has deployed AI-powered health coaching apps that analyze user behavior and provide personalized recommendations, resulting in a 32% improvement in chronic disease management metrics among users.
For healthcare businesses, preventative applications offer compelling economics:
- Reduced hospitalization costs
- Lower emergency care utilization
- New revenue streams through wellness services
- Improved patient retention and satisfaction
Technology companies like Apple and Fitbit are partnering with insurers and healthcare providers to create ecosystems where wearable data informs clinical decisions. These partnerships represent new business models where value is created through data-driven preventative care rather than treatment of established conditions.
Early Disease Detection
AI excels at pattern recognition within complex datasets, making it exceptionally effective at detecting subtle disease indicators that human clinicians might miss.
In oncology, AI systems now review mammograms with 99% accuracy while reducing false positives by 30%. This translates to earlier cancer detection, simpler treatments, better survival rates, and significantly lower treatment costs.
Similarly, AI algorithms analyzing retinal scans can detect diabetic retinopathy, cardiovascular disease risk, and early signs of Alzheimer's—often years before conventional diagnosis would be possible.
The business implications are substantial:
- Earlier interventions reduce overall treatment costs by 40-60%
- Screening services create new revenue opportunities
- Precision in early detection reduces unnecessary follow-up procedures
- Population health management becomes more effective and economical
A Singapore-based healthcare group reported 28% cost savings after implementing AI-powered early detection protocols for diabetes complications, while simultaneously improving patient outcomes.
Advanced Diagnostics
AI systems are increasingly functioning as diagnostic partners for clinicians, analyzing medical images, laboratory results, genomic data, and electronic health records to suggest potential diagnoses.
Systems like IBM's Watson for Health and Google's DeepMind can process and synthesize vast amounts of medical literature and clinical data far beyond human capabilities. This cognitive computing approach ensures diagnostic decisions are informed by the latest research and similar case histories.
In radiology, AI doesn't just flag abnormalities—it quantifies them, tracks changes over time, and correlates findings across multiple imaging modalities. The result is more accurate, consistent, and comprehensive diagnostic information.
For healthcare organizations, the business benefits include:
- Reduced diagnostic errors (currently estimated to cost $750 billion annually worldwide)
- Faster turnaround times increasing facility throughput and capacity
- Enhanced specialist productivity by filtering and prioritizing cases
- Improved clinical documentation supporting better reimbursement
A study at the National University Hospital in Singapore found that integrating AI diagnostic support reduced diagnostic costs by 23% while improving accuracy by 17%—a dual benefit rarely achieved with other healthcare interventions.
Clinical Decision Support
Beyond diagnosis, AI systems now support treatment planning and clinical decision-making throughout the care journey. These systems analyze patient-specific data against treatment protocols, research evidence, and outcomes from similar cases to recommend optimal interventions.
Predictive analytics models can identify patients at risk for complications, readmissions, or treatment non-response, enabling proactive management strategies. For example, an AI system at Singapore General Hospital predicted sepsis onset in ICU patients up to six hours earlier than traditional methods, reducing mortality by 18%.
For healthcare executives, clinical decision support systems offer multiple value propositions:
- Standardization of care leading to better outcomes and lower costs
- Reduced variation in practice patterns
- Faster adoption of evidence-based practices
- Better management of clinical risk
Implementing clinical decision support requires thoughtful integration with clinical workflows, provider education, and continuous validation. Organizations that execute effectively gain both quality and efficiency advantages.
Treatment and Care Delivery
Robotics and AI are fundamentally changing how care is delivered across healthcare settings. Surgical robots like the da Vinci system enable minimally invasive procedures with greater precision, while care delivery robots support medication administration, patient monitoring, and logistics.
In rehabilitation, robotic exoskeletons assist patients with mobility limitations, providing consistent therapy that adapts to patient progress. Meanwhile, AI-powered remote monitoring systems enable hospital-at-home models that reduce costs while improving patient satisfaction.
The business case for these technologies centers on:
- Improved clinical outcomes leading to better reimbursement under value-based care
- Reduced length of stay increasing facility capacity
- Lower complication rates decreasing costs
- Enhanced ability to care for complex patients
Raffles Medical Group in Singapore reported that robotic surgical systems increased their procedural capacity by 22% while reducing recovery times by 35%, demonstrating how technology investments can drive both clinical and business results.
End-of-Life Care Solutions
As populations age, particularly in developed economies like Singapore, end-of-life care presents growing challenges and opportunities. AI and robotics are creating more dignified, personalized, and economically sustainable approaches to this sensitive life stage.
Companion robots like PARO (a therapeutic seal robot) provide comfort and cognitive stimulation for dementia patients, reducing agitation and the need for sedating medications. AI monitoring systems allow elderly individuals to remain independent longer while ensuring safety through fall detection and behavior analysis.
Virtual reality experiences developed at Singapore's Centre for Healthcare Innovation provide pain management and emotional support for terminal patients, reducing medication requirements and improving quality of life.
For healthcare organizations, these technologies address critical business challenges:
- Extending limited caregiver resources
- Reducing costly emergency interventions
- Creating scalable models for elder care
- Providing more humane alternatives to institutional care
The economic imperative is clear: traditional models of end-of-life care are unsustainable given demographic trends. AI and robotics offer pathways to better care at lower costs, representing both ethical and business advantages.
Research and Drug Development
AI is dramatically accelerating pharmaceutical research and development, addressing an innovation process that traditionally costs billions and takes over a decade per successful drug.
Machine learning algorithms now predict molecular properties, identify potential drug candidates, optimize dosing regimens, and even repurpose existing medications for new indications. This computational approach is estimated to reduce early-stage drug development timelines by 40-50%.
In clinical trials, AI improves protocol design, participant selection, and data analysis while enabling more adaptive trial methodologies. Singapore's recent establishment of AI-powered clinical trial matching platforms has increased trial enrollment rates by 67% while reducing recruitment costs.
For healthcare and pharmaceutical executives, AI in research represents opportunities for:
- Faster time-to-market for new therapies
- Development of previously uneconomical treatments for rare conditions
- More precise targeting of therapies to patient subgroups
- Higher success rates in clinical development
These advantages are creating new partnership models between technology companies, research institutions, healthcare providers, and pharmaceutical manufacturers—reshaping the innovation ecosystem across Southeast Asia and globally.
Medical Training and Education
AI-powered simulation technologies are transforming how healthcare professionals develop and maintain clinical skills. Virtual patients respond naturally to treatment decisions, display realistic symptoms, and provide immediate feedback on clinical reasoning.
Surgical simulators using haptic feedback and AI analysis help surgeons practice complex procedures before attempting them on patients. These systems adapt to the learner's skill level, focusing on areas needing improvement and accelerating proficiency development.
At the Business+AI Masterclass, healthcare leaders learn how these technologies can address workforce development challenges in ways traditional education cannot match:
- Consistent training experiences regardless of location
- Objective assessment of clinical competency
- Training for rare conditions or emergency scenarios
- Rapid skill development for new procedures or technologies
Singapore's healthcare institutions have pioneered these approaches, reporting 40% faster skill acquisition and better retention compared to traditional training methods.
Implementation Challenges and Solutions
Despite compelling benefits, healthcare organizations face significant challenges when implementing AI and robotics. Understanding these barriers—and strategies to overcome them—is essential for success.
Data Integration and Quality
AI systems require access to high-quality, integrated data from disparate sources. Many healthcare organizations struggle with fragmented systems, inconsistent data formats, and incomplete information.
Successful organizations address this by:
- Creating dedicated data governance frameworks
- Implementing data lakes that standardize information from multiple sources
- Establishing data quality monitoring and improvement processes
- Developing clear data sharing agreements with technology partners
Workforce Adoption and Change Management
Clinician resistance remains a primary barrier to AI implementation. Healthcare professionals may fear displacement, distrust algorithmic recommendations, or resist workflow changes.
Effective change management strategies include:
- Early clinician involvement in technology selection and implementation
- Transparent communication about AI's role as augmenting rather than replacing expertise
- Phased implementation approaches with visible quick wins
- Continuous education and skill development opportunities
At Business+AI Forums, healthcare leaders share implementation experiences and best practices for addressing these human factors effectively.
Technical Infrastructure
AI and robotics often require significant computing resources, network capabilities, and specialized facilities. Organizations must plan for these infrastructure requirements to ensure successful deployment.
Leading organizations address infrastructure challenges through:
- Cloud-based deployment models reducing on-premises requirements
- Phased technology implementation aligned with infrastructure upgrades
- Strategic partnerships with technology providers offering managed services
- Clear assessment of infrastructure requirements during planning phases
Return on Investment Realization
Quantifying returns from AI investments can be challenging, particularly when benefits manifest across multiple departments or over extended timeframes. Organizations often struggle to maintain support for initiatives when ROI is not immediately apparent.
Successful approaches include:
- Developing comprehensive metrics beyond direct financial returns
- Establishing baseline measurements before implementation
- Setting realistic timelines for benefit realization
- Creating balanced scorecards incorporating clinical, operational, and financial outcomes
The Business+AI Consulting team specializes in developing these measurement frameworks for healthcare organizations implementing AI technologies.
Regulatory and Ethical Considerations
The regulatory landscape for healthcare AI and robotics continues to evolve rapidly. Organizations must navigate complex requirements while addressing legitimate ethical concerns about these technologies.
Regulatory Compliance
Singapore's Health Sciences Authority has developed specific frameworks for AI as a medical device, while the Personal Data Protection Act governs health data usage. Similar regulations exist across Southeast Asia, though with significant variation.
Organizations implementing AI must:
- Conduct thorough regulatory assessments for each technology
- Implement validation processes meeting regulatory standards
- Maintain documentation of system performance and decision logic
- Establish clear processes for managing regulatory changes
Ethical Implementation
Beyond regulatory compliance, organizations must address ethical dimensions of healthcare AI:
- Algorithmic Bias: Ensuring AI systems perform consistently across different patient populations
- Transparency: Making algorithmic decision processes understandable to clinicians and patients
- Accountability: Establishing clear responsibility frameworks for AI-assisted decisions
- Privacy: Protecting sensitive health information while enabling beneficial data usage
Leaders addressing these challenges effectively typically establish AI ethics committees including diverse stakeholders and implement formal assessment frameworks for evaluating new technologies.
The Path Forward
For healthcare executives, the transformative potential of AI and robotics is clear, but the path to successful implementation requires strategic planning and execution. Based on successful implementations across Southeast Asia, a practical roadmap emerges:
1. Strategic Assessment and Prioritization
Begin by identifying specific organizational challenges where AI offers the greatest potential value. Prioritize opportunities based on:
- Alignment with strategic objectives
- Potential impact on clinical and business outcomes
- Implementation complexity and resource requirements
- Organizational readiness for adoption
2. Build Internal Capabilities
Successful AI implementation requires new organizational capabilities. Develop these through:
- Identifying internal champions across clinical and operational departments
- Creating multidisciplinary AI governance structures
- Investing in staff education about AI capabilities and limitations
- Establishing data science capabilities through hiring or partnerships
3. Partner Selection
Few healthcare organizations can implement AI solutions independently. Effective partner selection involves:
- Evaluating potential partners' healthcare domain expertise
- Assessing alignment between partner solutions and specific use cases
- Confirming partners' regulatory compliance and security practices
- Establishing clear intellectual property and data ownership agreements
4. Phased Implementation
Successful organizations typically begin with limited-scope implementations that demonstrate value before scaling. This phased approach includes:
- Pilot projects with clearly defined success metrics
- Validation of results against current practices
- Refinement of implementation based on early learnings
- Gradual expansion to additional use cases or departments
5. Continuous Evaluation and Optimization
High-performing AI implementations require ongoing management, not just initial deployment. Establish processes for:
- Regular performance monitoring against defined metrics
- Continuous model validation and updating
- User feedback collection and incorporation
- Assessment of emerging technologies for additional opportunities
Through Business+AI Workshops, healthcare leaders can develop comprehensive implementation plans tailored to their organizations' specific needs and constraints.
Conclusion
The integration of AI and robotics into healthcare represents more than technological advancement—it's a fundamental transformation of how care is delivered, how diseases are prevented and treated, and how healthcare organizations operate.
For business leaders and healthcare executives, particularly in Singapore and Southeast Asia, this transformation offers extraordinary opportunities to address pressing challenges: aging populations, rising costs, workforce limitations, and increasing consumer expectations.
The organizations that will thrive in this new era share common characteristics: they approach AI strategically rather than tactically, they build both technological infrastructure and human capabilities, they address ethical and regulatory considerations proactively, and they implement with discipline and patience.
The technologies discussed in this article—from diagnostic AI to surgical robotics, from predictive analytics to virtual training environments—all serve a common purpose: creating healthcare systems that deliver better care to more people at sustainable costs. This goal aligns perfectly with both business imperatives and healthcare's fundamental mission.
As you consider your organization's journey toward AI-enabled healthcare, remember that implementation success depends not just on selecting the right technologies, but on creating the right organizational conditions for innovation and adoption. The path may be challenging, but the potential rewards—in clinical outcomes, operational efficiency, and business performance—make it a journey worth taking.
Ready to transform your healthcare organization with AI and robotics? Join the Business+AI Membership program to access expert guidance, implementation workshops, and a community of innovative healthcare leaders who are successfully navigating this technological revolution. Turn AI potential into tangible business results with Business+AI.