Revolutionizing Finance and Reporting with AI Agents: Implementation Guide for APAC Businesses
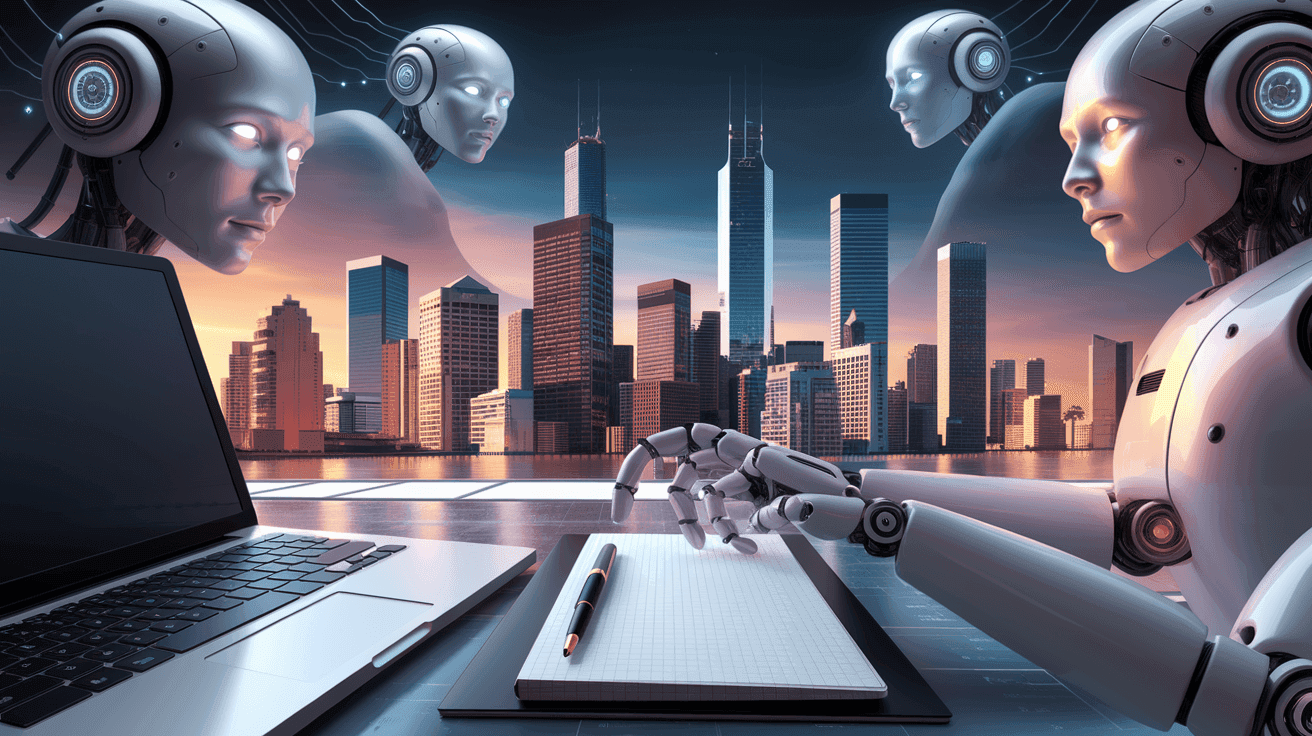
- Understanding AI Agents in Finance and Reporting
- Key Applications of AI Agents in Modern Finance Departments
- Implementation Framework for Finance AI Agents
- Overcoming Challenges in AI Agent Adoption
- Building a Roadmap for AI-Powered Finance Operations
- Case Studies: AI Agents Success Stories in APAC
- Conclusion: Taking the Next Step in Your AI Journey
Finance departments across APAC are undergoing a silent revolution. Beyond simple automation, a new generation of AI-powered digital workers—AI agents—are transforming how financial operations, analysis, and reporting are performed. These intelligent systems can work autonomously to complete complex tasks, learn from interactions, and deliver unprecedented efficiency while maintaining compliance and accuracy. As finance leaders face mounting pressure to deliver more insights with fewer resources, AI agents offer a compelling solution that goes beyond traditional automation tools.
For businesses in Singapore and across APAC, where financial complexity often intersects with rapid digital transformation, AI agents represent not just an incremental improvement but a fundamental shift in how finance functions operate. This article explores how these sophisticated AI systems are specifically reshaping finance and reporting processes, providing an implementation roadmap tailored to the unique challenges and opportunities in the region.
Understanding AI Agents in Finance and Reporting
AI agents represent a significant evolution beyond basic automation tools. Unlike traditional finance software that follows rigid, predefined rules, AI agents can perceive their environment, make decisions based on specific goals, and take autonomous actions—all while learning and improving over time. In the finance context, this translates to systems that can understand financial documents, extract relevant information, reconcile discrepancies, generate reports, and even provide decision recommendations with minimal human intervention.
What distinguishes true AI agents from other AI implementations is their ability to execute end-to-end processes rather than just individual tasks. They combine multiple AI capabilities—natural language processing to understand documents and queries, machine learning to identify patterns and anomalies, and decision intelligence to determine appropriate actions. When applied to finance operations, these agents can transform disjointed workflows into seamless processes that operate continuously and adapt to changing conditions.
The technology stack supporting finance AI agents typically includes:
- Large language models for understanding and generating financial text
- Computer vision for processing financial documents and receipts
- Specialized financial models trained on accounting principles
- Integration APIs to connect with existing finance systems
- Orchestration layers to coordinate multiple agents across processes
- Security and governance frameworks to ensure compliance
The sophistication of these agents continues to increase, with the latest generation capable of handling complex judgment calls that previously required experienced finance professionals. While they don't replace human expertise, they dramatically amplify it—allowing finance teams to focus on strategic analysis rather than routine processing.
Key Applications of AI Agents in Modern Finance Departments
AI agents are finding diverse applications across finance functions, delivering value through specialized capabilities tailored to financial processes:
Financial Data Aggregation and Reconciliation
Finance departments typically draw data from multiple systems—ERP platforms, banking portals, expense management tools, and subsidiary ledgers. AI agents can autonomously collect this information, standardize formats, identify and resolve discrepancies, and prepare reconciled datasets for analysis or reporting. This reduces a process that often consumed days to hours or even minutes, while significantly improving accuracy.
In multinational organizations with operations across APAC, these agents can also navigate multi-currency transactions, different accounting standards (IFRS vs. local GAAP), and region-specific reporting requirements without manual intervention.
Automated Reporting and Disclosure Preparation
Periodic financial reporting remains one of the most resource-intensive processes for finance teams. AI agents can transform this workflow by automatically generating financial statements, management reports, and regulatory disclosures from underlying data. They can ensure consistency across documents, apply the correct accounting treatments, and even draft explanatory notes for significant items.
For public companies, these agents can assist with SGX, HKEX, or other exchange-specific disclosure requirements, ensuring compliance while reducing preparation time. They can also generate first drafts of MD&A sections by analyzing performance trends and highlighting material changes.
Financial Analysis and Anomaly Detection
Beyond basic reporting, AI agents excel at continuous financial analysis—monitoring transactions, identifying patterns, and flagging anomalies that might indicate errors, fraud, or business opportunities. Unlike traditional rule-based systems, these agents can detect subtle patterns that would escape predefined rules, adapting their analysis as financial behaviors evolve.
For instance, an AI agent might identify that a particular expense category has increased 40% above seasonal norms, automatically investigate contributing factors, and prepare a summary analysis for the finance team. This proactive monitoring helps organizations address issues before they impact financial statements.
Risk Assessment and Compliance Monitoring
Regulatory compliance represents a significant burden for finance departments, particularly in highly regulated industries common across APAC. AI agents can continuously monitor transactions against regulatory requirements, company policies, and industry standards, alerting teams to potential compliance issues.
These agents can track changing regulations across multiple jurisdictions, assess the impact on financial processes, and recommend adjustments to maintain compliance. This capability is particularly valuable for organizations operating across multiple APAC countries with divergent regulatory environments.
Real-time Financial Insights and Forecasting
Traditional financial forecasting often relies on quarterly or monthly cycles, limiting organizations' ability to respond to rapidly changing conditions. AI agents enable continuous forecasting—constantly updating projections based on the latest transactions, market conditions, and operational data.
These systems can generate scenario analyses, stress tests, and sensitivity models that help finance leaders understand potential outcomes and make more informed decisions. The real-time nature of these insights is particularly valuable in volatile markets or during major business transitions.
Tax Preparation and Planning
Tax compliance and optimization represent significant opportunities for AI agent deployment. These systems can interpret complex tax codes across multiple jurisdictions, identify applicable treatments, prepare required filings, and even suggest optimization strategies.
For businesses operating across the diverse tax environments of APAC countries, this capability can dramatically reduce compliance costs while improving accuracy and minimizing tax risks.
Implementation Framework for Finance AI Agents
Successfully implementing AI agents in finance requires a structured approach that balances technological capabilities with operational realities:
Assessment and Opportunity Identification
Begin by mapping your finance processes to identify high-value opportunities for AI agent deployment. The ideal candidates typically share several characteristics:
- High volume of standardized transactions or documents
- Significant manual effort currently required
- Clear rules and patterns, even if complex
- Measurable outcomes and success criteria
- Moderate to high business impact
Common starting points include accounts payable processing, expense management, financial close activities, and management reporting. Prioritize opportunities based on potential impact, implementation complexity, and alignment with strategic priorities.
Data Infrastructure Requirements
Finance AI agents rely on high-quality, accessible data. Before implementation, organizations should assess their data infrastructure against several key requirements:
- Data completeness and quality across source systems
- Standardization of financial data formats and definitions
- Integration capabilities with existing finance platforms
- Historical data availability for agent training
- Data governance and security controls
Many organizations find they need to enhance their data infrastructure before fully deploying AI agents. This foundational work, while sometimes challenging, creates value beyond the immediate AI implementation by improving overall financial data management.
Integration with Existing Finance Systems
Most organizations have significant investments in financial systems like ERPs, accounting software, and reporting tools. Effective AI agent implementation requires thoughtful integration with these existing platforms through:
- API connections to core financial systems
- Secure access management for data retrieval and updates
- Workflow integration for handoffs between systems and agents
- Exception handling mechanisms when agent capabilities are exceeded
- Audit trails that document agent actions within the broader system
A well-designed integration strategy preserves existing investments while enhancing capabilities through AI agents that complement rather than replace core financial systems.
Governance and Control Mechanisms
Finance operations require strict controls to ensure accuracy, compliance, and security. AI agent implementations must incorporate appropriate governance mechanisms:
- Clear ownership of agent oversight and performance
- Defined approval workflows for agent-generated outputs
- Monitoring systems that track agent actions and decisions
- Regular validation of agent performance against standards
- Escalation procedures for exceptions and errors
- Periodic reviews of agent logic and decision processes
These controls ensure that while agents operate autonomously, they remain aligned with organizational standards and requirements.
Responsible AI Considerations Specific to Finance
Finance applications of AI require particular attention to responsible implementation practices:
- Explainability of financial decisions and recommendations
- Bias detection and mitigation in financial analyses
- Compliance with relevant regulations (MAS Guidelines on AI, etc.)
- Privacy protection for sensitive financial information
- Appropriate human oversight for material financial processes
- Transparent documentation of AI methodologies
Organizations should develop specific responsible AI standards for finance applications that reflect both general best practices and finance-specific requirements.
Training and Deployment Approach
Successful deployment typically follows a phased approach:
- Pilot implementation with limited scope and close monitoring
- Validation of results against established standards
- Gradual expansion of agent responsibilities and autonomy
- Continuous performance monitoring and improvement
- Regular retraining with new data and scenarios
This measured approach builds confidence in the technology while allowing time for organizational adaptation and system refinement.
Overcoming Challenges in AI Agent Adoption
While the potential benefits are substantial, organizations frequently encounter several challenges when implementing AI agents in finance:
Data Quality and Standardization Hurdles
Finance data often resides in disparate systems with inconsistent formats, definitions, and quality standards. AI agents require clean, standardized data to function effectively.
Solution approach: Begin with a focused data quality initiative in the specific domains targeted for agent implementation. Create standardized data dictionaries, implement validation rules, and establish ongoing data governance practices. Consider deploying specialized data preparation agents as a first step before implementing more advanced finance agents.
Managing Complexity in Financial Regulations
Financial reporting and compliance operate under complex, changing regulatory frameworks that vary across APAC jurisdictions. AI agents must navigate these requirements while maintaining compliance.
Solution approach: Develop specialized compliance modules that are regularly updated with regulatory changes. Partner with regulatory experts to validate agent logic and incorporate appropriate controls. Consider beginning with less regulated processes while building capabilities for more complex regulatory environments.
Change Management and Team Adaptation
Finance professionals may be concerned about how AI agents will affect their roles and responsibilities. Successful implementation requires thoughtful change management.
Solution approach: Involve finance team members early in the process, focusing on how agents will enhance rather than replace their work. Provide training on collaborating with AI agents and develop clear guidelines for the division of responsibilities. Showcase early wins to build confidence in the technology.
Security and Privacy Considerations
Financial data is highly sensitive, creating security and privacy challenges for AI agent implementations.
Solution approach: Implement end-to-end encryption for agent communications, strict access controls, and comprehensive audit trails. Ensure compliance with relevant privacy regulations (PDPA in Singapore, etc.) and consider data residency requirements when designing agent architectures.
Cost-Benefit Analysis and ROI Measurement
Organizations often struggle to accurately forecast and measure the financial impact of AI agent implementations.
Solution approach: Develop a comprehensive ROI framework that considers both direct cost savings (labor reduction, error prevention) and indirect benefits (faster reporting, improved decision-making, risk reduction). Start with pilots that have clearly measurable outcomes to build an evidence base for larger investments.
Integration with Legacy Systems
Many finance departments rely on legacy systems that may not readily support modern AI integrations.
Solution approach: Consider middleware solutions that can bridge modern AI capabilities with legacy platforms. Develop phased modernization plans that align system upgrades with AI implementation. In some cases, use AI agents to extract and transform data from legacy systems as an intermediate solution while planning longer-term system modernization.
Building a Roadmap for AI-Powered Finance Operations
A successful transition to AI-enhanced finance operations requires a clear roadmap that balances ambition with pragmatism:
Starting with High-Impact, Low-Risk Use Cases
Begin your AI journey with applications that offer significant benefits while minimizing implementation risks. Ideal starting points typically include:
- Accounts payable automation with intelligent document processing
- Expense report auditing and processing
- Account reconciliation and variance analysis
- Standard management reporting
- Financial data validation and cleaning
These applications provide visible wins that build momentum while developing organizational capabilities for more complex implementations.
Phased Implementation Approach
Rather than attempting a comprehensive transformation, most successful organizations follow a staged approach:
Phase 1: Foundation Building (3-6 months)
- Deploy initial AI agents for well-defined, limited-scope processes
- Establish governance framework and performance monitoring
- Develop team capabilities through training and hands-on experience
- Measure results and refine implementation approach
Phase 2: Expansion and Integration (6-12 months)
- Extend successful agent implementations to related processes
- Begin connecting multiple agents into coordinated workflows
- Deepen integration with core financial systems
- Enhance agent capabilities through additional training
Phase 3: Transformation (12-24 months)
- Implement more complex, judgment-intensive applications
- Deploy agent networks that coordinate across finance functions
- Develop predictive and prescriptive capabilities
- Redesign finance processes around AI capabilities
This measured approach allows organizations to build capabilities while delivering incremental value throughout the journey.
Skills Development and Team Structure
As AI agents take on more routine tasks, finance teams need to evolve their capabilities. Key focus areas include:
- Developing "AI collaboration skills"—knowing how to effectively work with AI agents
- Strengthening analytical and interpretive capabilities
- Building technical literacy to understand and guide agent development
- Enhancing strategic and business partnership skills
Many organizations are creating hybrid roles that combine financial expertise with technical knowledge, serving as bridges between traditional finance functions and AI implementation teams.
Measuring Success and Iterating
Establish clear success metrics that align with strategic objectives:
- Efficiency metrics: Process time reduction, capacity creation, cost savings
- Quality metrics: Error reduction, compliance improvement, accuracy rates
- Strategic metrics: Insight generation, decision speed, business impact
Regularly review these metrics and use the insights to refine your implementation approach, adjusting priorities and resources as you learn.
Scaling Across Finance Functions
As initial implementations prove successful, expand your AI agent deployment across the finance organization. Consider both horizontal scaling (applying similar agents to additional business units or geographies) and vertical scaling (deploying more sophisticated agents for complex processes).
Create reusable components and templates that accelerate implementation, and develop centers of excellence that share best practices across the organization.
Future-Proofing Your Finance AI Strategy
The capabilities of AI agents continue to evolve rapidly. Build flexibility into your strategy through:
- Modular architecture that allows component upgrades
- Regular reassessment of available technologies
- Partnerships with AI providers focused on finance applications
- Internal innovation programs that explore emerging capabilities
Maintain a balance between leveraging current capabilities and preparing for future advancements that may fundamentally reshape finance operations.
Case Studies: AI Agents Success Stories in APAC
Multinational Corporation Streamlining Financial Reporting
A Singapore-headquartered multinational with operations across 12 APAC countries implemented AI agents to transform their financial close and reporting process. The company faced significant challenges with their monthly close, including:
- Data collection from multiple ERP systems and local ledgers
- Reconciliation across currencies and accounting standards
- Complex elimination entries for intercompany transactions
- Compliance with both group and local reporting requirements
By implementing a network of specialized AI agents, they reduced their close process from 12 days to 5 days while improving accuracy and compliance. The agents automatically collected and standardized data, identified and resolved discrepancies, generated elimination entries, and produced draft financial statements for review.
Key success factors included:
- Starting with data standardization across source systems
- Developing specialized agents for specific close activities
- Implementing robust validation controls
- Maintaining clear human oversight for material judgments
- Gradual expansion of agent responsibilities as confidence grew
The finance team now focuses primarily on analysis and decision support rather than data processing, creating significantly more value for the business.
Banking Institution Implementing Compliance Agents
A regional bank based in Singapore deployed AI agents to manage regulatory compliance across its operations in five countries. The bank faced mounting compliance costs and risks due to rapidly evolving regulations and increasing reporting requirements.
Their implementation included:
- Regulatory monitoring agents that track changes across jurisdictions
- Transaction surveillance agents that identify potential compliance issues
- Reporting agents that automate regulatory filings
- Documentation agents that maintain compliance evidence
These agents reduced compliance costs by 35% while improving accuracy and reducing regulatory risks. By automating routine compliance activities, the bank redirected resources toward strategic risk management and customer-focused initiatives.
The implementation succeeded by:
- Partnering closely with compliance experts to encode regulatory requirements
- Building in explainability for all agent decisions
- Implementing comprehensive audit trails
- Starting with less complex regulations before tackling more nuanced requirements
- Creating clear escalation paths for complex scenarios
Mid-Sized Company Automating Tax Reporting
A growing technology company with operations in Singapore, Malaysia, and Indonesia implemented AI agents to manage their increasingly complex tax compliance obligations. The company was struggling with:
- Different tax regimes across countries
- Frequent changes to tax regulations
- Limited in-house tax expertise
- Time-consuming tax preparation processes
Their solution deployed specialized tax agents that:
- Monitored tax law changes across jurisdictions
- Extracted relevant transaction data from financial systems
- Applied appropriate tax treatments based on current regulations
- Prepared draft tax filings for review
- Identified potential tax planning opportunities
The implementation reduced tax preparation time by 60% while improving compliance and identifying tax savings. The finance team gained confidence in their tax processes despite operating across multiple complex jurisdictions.
Key lessons from their experience include:
- The importance of high-quality source data
- The need for regular updates to tax knowledge bases
- The value of maintaining human review for material tax positions
- The benefits of starting with simpler tax processes before tackling more complex areas
Conclusion: Taking the Next Step in Your AI Journey
The transformation of finance and reporting through AI agents represents one of the most significant opportunities for finance leaders in APAC. These intelligent systems are not merely automating existing processes—they're enabling fundamentally new approaches to financial operations that combine unprecedented efficiency with enhanced insight generation.
Organizations that successfully implement AI agents in finance are gaining multiple competitive advantages:
- Faster access to financial insights that drive better business decisions
- Reduced costs for routine financial operations
- Enhanced compliance with complex regulatory requirements
- Greater capacity for value-added analysis and business partnership
- Improved financial accuracy and reduced risks
However, this transformation requires thoughtful implementation that balances technological possibilities with organizational realities. The most successful organizations approach AI adoption as a strategic journey rather than a series of tactical projects, building capabilities and delivering value at each step.
For finance leaders in Singapore and across APAC, the question is no longer whether to implement AI agents but how to do so most effectively. Those who move forward with clear strategy, appropriate governance, and a focus on business outcomes will position their organizations for success in an increasingly competitive landscape.
The journey toward AI-enhanced finance operations is complex but manageable with the right approach and partners. By starting today with well-defined initiatives and building capabilities systematically, finance leaders can transform their operations while creating significant value for their organizations.
Ready to explore how AI agents can transform your finance and reporting processes? Join the Business+AI ecosystem to connect with experts, learn from successful implementations, and develop your organization's AI strategy.
Explore our membership benefits to gain access to exclusive resources, workshops, and a community of leaders navigating similar transformation journeys.
Attend our specialized AI for Finance workshops or masterclasses to develop practical implementation plans tailored to your organization's specific needs.
Connect with our community of finance and technology leaders at the upcoming Business+AI Forum to share experiences and insights on the future of finance.
For organizations seeking more personalized guidance, our consulting services provide expert support throughout your AI implementation journey.