Managing AI Risks: A Practical Checklist for Singaporean Companies
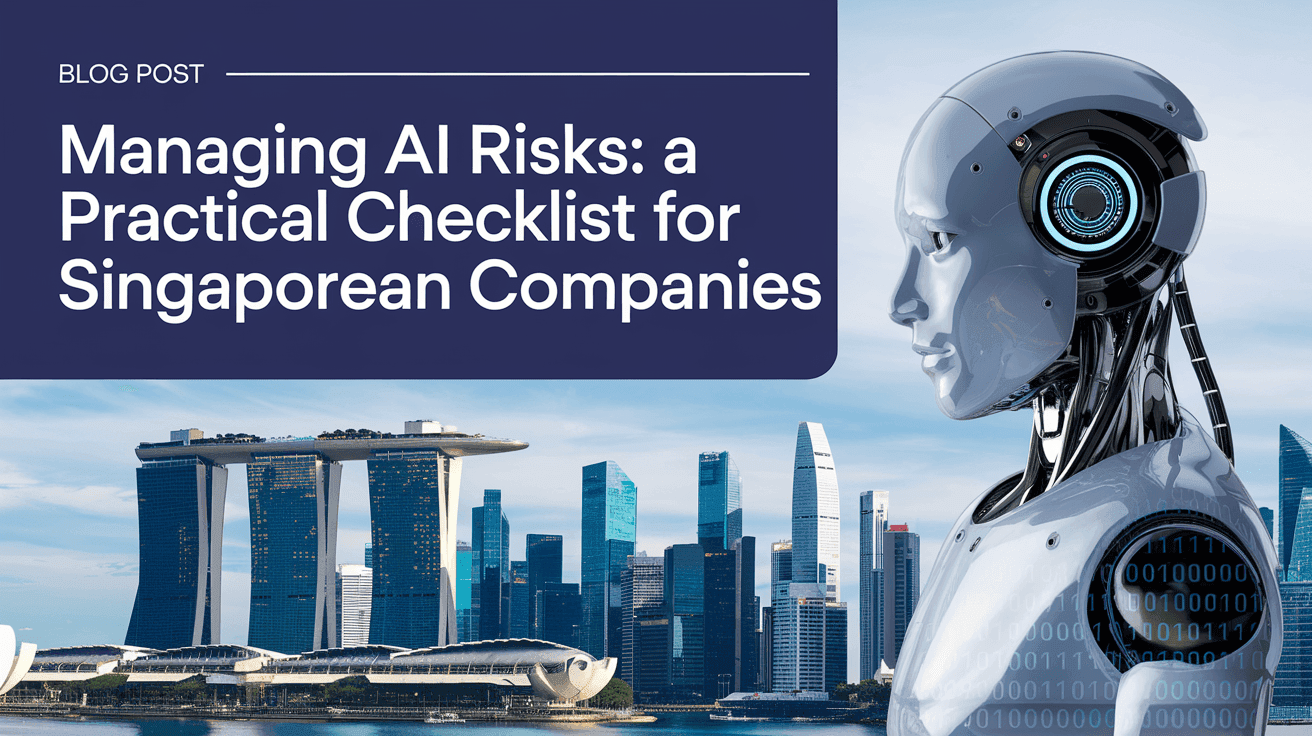
- Introduction
- Understanding AI Risks in the Singaporean Context
- Risk Category 1: Governance and Accountability
- Risk Category 2: Ethical and Responsible AI Use
- Risk Category 3: Technical Robustness and Security
- Risk Category 4: Data Governance and Privacy
- Risk Category 5: Business and Operational Considerations
- Implementation Roadmap
- Conclusion
Artificial Intelligence (AI) presents tremendous opportunities for Singaporean businesses across sectors. From automating routine tasks to enabling data-driven decision-making, AI technologies can drive efficiency, innovation, and competitive advantage. However, these opportunities come with significant risks that require careful management, especially in Singapore's highly regulated business environment.
As Singapore positions itself as an AI hub in Asia, companies face increasing pressure to adopt AI responsibly. The Singapore government has introduced frameworks like the Model AI Governance Framework and initiatives like AI Verify to guide organizations. Yet many businesses struggle to translate these guidelines into practical implementation steps.
This article provides a comprehensive checklist for Singaporean companies to effectively manage AI risks while maximizing the benefits of AI adoption. By following this structured approach, organizations can navigate the complex landscape of AI governance, ethics, technical considerations, data privacy, and operational challenges specific to Singapore's business context.
Understanding AI Risks in the Singaporean Context
Singapore has taken a proactive approach to AI governance, establishing itself as a leader in ethical AI implementation in Asia. The Personal Data Protection Commission (PDPC) of Singapore has developed the Model AI Governance Framework, which provides detailed guidance on deploying AI responsibly. Additionally, the AI Verify Foundation offers tools for organizations to validate their AI systems.
For Singaporean businesses, AI risk management must account for:
- Singapore's emphasis on fairness, ethics, accountability, and transparency (FEAT) principles
- The high standards of data protection under the Personal Data Protection Act (PDPA)
- Sector-specific regulations (e.g., MAS guidelines for financial services)
- The multicultural nature of Singapore's workforce and customer base
- The city-state's strategic position as a business and technology hub in Southeast Asia
Common AI implementation challenges for Singaporean companies include securing AI expertise in a competitive talent market, managing cross-border data flows within regional operations, addressing bias in AI systems across diverse cultural contexts, and balancing innovation with compliance.
Risk Category 1: Governance and Accountability
Establishing robust governance structures is fundamental to managing AI risks effectively. This includes:
Establishing an AI Governance Committee
Form a cross-functional team with representatives from IT, legal, risk management, business units, and executive leadership. This committee should meet regularly to review AI initiatives, assess risks, and ensure alignment with organizational values and regulatory requirements.
Defining Clear Roles and Responsibilities
Document specific accountabilities for:
- AI system owners responsible for overall performance
- Data scientists and engineers building the systems
- Business stakeholders using the outputs
- Risk and compliance officers providing oversight
- Executive sponsors making strategic decisions
Implementing Documentation Protocols
Create comprehensive documentation covering:
- AI system design decisions and justifications
- Risk assessments conducted throughout development
- Testing procedures and results
- Deployment criteria and approvals
- Ongoing monitoring metrics
Ensuring Board-Level Visibility
Establish a reporting framework that brings critical AI risks to board attention. Regular updates should include risk metrics, notable incidents, and significant changes to AI systems or the regulatory environment.
Risk Category 2: Ethical and Responsible AI Use
Singapore's approach to AI emphasizes human-centricity and ethical considerations. Companies should:
Align with Singapore's AI Ethics Principles
Develop an AI ethics policy that incorporates Singapore's FEAT principles, ensuring systems are designed to be fair, ethical, accountable, and transparent. Review this policy regularly to reflect evolving standards and stakeholder expectations.
Implement Bias Detection and Mitigation
Establish processes to:
- Evaluate training data for potential biases relevant to Singapore's multicultural context
- Test AI systems for disparate outcomes across different demographic groups
- Apply mitigation techniques when bias is detected
- Document bias assessment methodologies and results
Ensure Explainability and Transparency
Develop appropriate explanations for different stakeholders:
- Technical documentation for internal teams
- Simplified explanations for business users
- Clear communications for customers affected by AI-driven decisions
- Regulatory-ready documentation that meets Singapore's requirements
Maintain Meaningful Human Oversight
Define clear procedures for:
- Human review of high-stakes AI decisions
- Override mechanisms when necessary
- Regular audits of automated processes
- Training for employees working alongside AI systems
Risk Category 3: Technical Robustness and Security
Technical reliability is crucial for risk management. Organizations should:
Establish Security Requirements
Implement security measures specific to AI systems:
- Access controls for training data and models
- Encryption for sensitive AI components
- Monitoring for unauthorized access or model extraction
- Integration with existing cybersecurity frameworks
Develop Testing Protocols
Create comprehensive testing regimes:
- Performance testing under various conditions
- Adversarial testing to identify vulnerabilities
- Integration testing with existing systems
- User acceptance testing with relevant stakeholders
Monitor for Drift and Degradation
Implement ongoing monitoring to detect:
- Data drift (changes in input data patterns)
- Concept drift (changes in the underlying relationships)
- Performance degradation over time
- Unexpected outputs or behaviors
Plan for Technical Failures
Develop contingency measures for when AI systems fail:
- Fallback mechanisms for critical functions
- Incident response procedures
- Business continuity arrangements
- Recovery protocols
Risk Category 4: Data Governance and Privacy
Data management is particularly important given Singapore's robust privacy regulations:
Comply with Singapore's PDPA
Ensure AI systems adhere to Singapore's data protection requirements:
- Obtain appropriate consent for data collection and use in AI
- Implement data protection impact assessments
- Enable data access and correction requests
- Maintain data breach response capabilities
Establish Data Quality Practices
Develop processes to ensure data quality:
- Data cleaning and validation protocols
- Metadata management
- Lineage tracking
- Regular data quality audits
Implement Data Minimization
Apply principles of data minimization:
- Collect only necessary data for AI functions
- Define data retention periods
- Anonymize or de-identify data where possible
- Implement secure data deletion processes
Manage Data Sharing Responsibly
Create frameworks for responsible data sharing:
- Data sharing agreements with vendors and partners
- Cross-border data transfer protocols
- Data classification systems
- Access controls based on need-to-know principles
Risk Category 5: Business and Operational Considerations
Integrating AI risk management into broader business operations is essential:
Assess and Manage Vendors
Develop vendor management processes for AI providers:
- Due diligence procedures for AI vendors
- Contractual requirements for risk management
- Ongoing monitoring of vendor performance
- Exit strategies for vendor relationships
Implement Change Management
Prepare the organization for AI-driven changes:
- Communication plans for affected stakeholders
- Training programs for employees working with AI
- Transition plans for processes being automated
- Feedback mechanisms for continuous improvement
Establish Business Continuity Plans
Prepare for potential disruptions:
- Backup plans for AI-dependent processes
- Redundancy measures for critical systems
- Regular testing of continuity arrangements
- Clear escalation procedures
Conduct Cost-Benefit Analysis
Implement regular review of AI investments:
- Tracking of realized benefits against expectations
- Assessment of ongoing costs and risks
- Analysis of alternative approaches
- Decision frameworks for continuing, modifying, or retiring AI systems
Implementation Roadmap
Implementing comprehensive AI risk management requires a phased approach:
Phase 1: Assessment (1-3 months)
- Inventory existing and planned AI systems
- Conduct risk assessments for each system
- Benchmark current practices against this checklist
- Identify gaps and prioritize actions
Phase 2: Foundation Building (3-6 months)
- Establish governance structures
- Develop key policies and procedures
- Implement essential technical controls
- Conduct initial training for key personnel
Phase 3: Implementation (6-12 months)
- Roll out comprehensive risk management measures
- Integrate with existing business processes
- Develop monitoring mechanisms
- Build internal capabilities
Phase 4: Maturity (Ongoing)
- Continuously improve based on experience
- Stay current with evolving regulations
- Benchmark against industry best practices
- Expand scope as AI adoption grows
Successful implementation requires executive sponsorship, adequate resources, and regular progress reviews. Companies that attended our Business+AI Forum report that establishing small cross-functional working groups with clear objectives tends to yield the best results during initial implementation phases.
Managing AI risks effectively is not just about compliance—it's about building sustainable competitive advantage through responsible innovation. For Singaporean companies, the journey toward safe and ethical AI implementation requires balancing multiple considerations: technical robustness, ethical principles, regulatory compliance, and business value.
By following the checklist outlined in this article, organizations can develop a structured approach to AI risk management that aligns with Singapore's forward-thinking governance frameworks while enabling business growth. As AI technologies continue to evolve rapidly, companies that establish strong risk management foundations today will be better positioned to adapt to tomorrow's challenges and opportunities.
Remember that AI risk management is not a one-time project but an ongoing process that requires continuous attention and refinement. By embedding risk considerations throughout the AI lifecycle, Singaporean businesses can build trust with customers, regulators, and other stakeholders while leveraging the transformative potential of artificial intelligence.
Navigating the complex landscape of AI risk management requires expertise and practical guidance. Business+AI offers comprehensive support for Singaporean companies implementing the checklist outlined in this article:
- Join our membership program to access exclusive resources, peer networking opportunities, and expert guidance on AI risk management.
- Attend our specialized workshops on AI governance and risk mitigation, tailored to Singapore's regulatory environment.
- Engage our consulting services for personalized assessment and implementation support.
- Participate in our masterclasses led by industry experts on specific aspects of AI risk management.
- Connect with solution providers and peers at our flagship annual Business+AI Forum to stay updated on emerging best practices.
Business+AI helps turn AI risk management theory into practical business reality. Contact us today to begin your journey toward responsible and effective AI implementation.