From Chatbots to Colleagues: The Shift to Agentic Co-workers
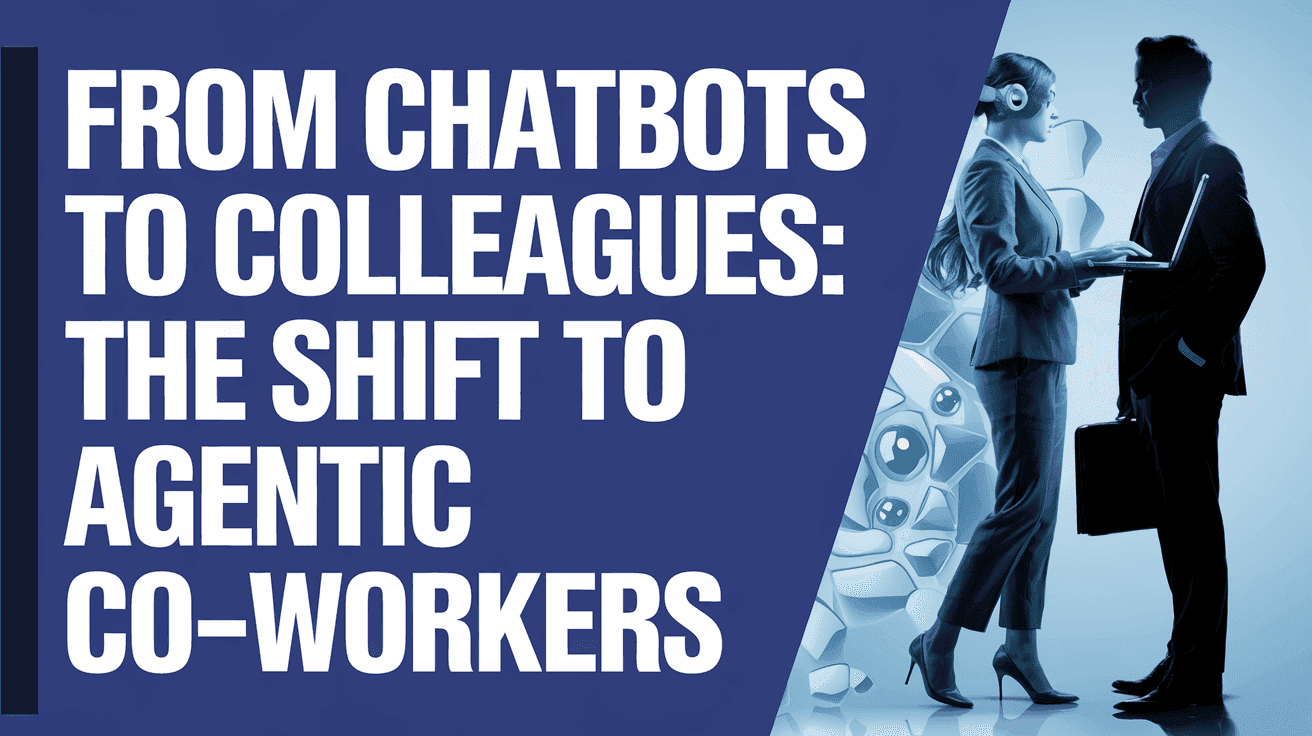
Table of Contents
- The Evolution from Reactive Tools to Proactive Partners
- Defining Agentic AI: What Makes These Systems Different
- Key Capabilities of AI Co-workers
- Real-World Applications Across Industries
- Implementation Challenges and Solutions
- The Human-AI Collaboration Model
- Measuring Business Impact and ROI
- Future Outlook: The Next Evolution
- Preparing Your Organization for Agentic AI
The business world is experiencing a profound transformation in how artificial intelligence integrates into the workplace. What began as simple chatbots with predetermined scripts and limited functionality is rapidly evolving into something much more sophisticated: agentic co-workers that function alongside humans as true digital colleagues.
This shift represents more than just a technological advancement—it signals a fundamental reimagining of the relationship between humans and machines in professional settings. As organizations worldwide transition from using AI as mere tools to embracing them as collaborative partners, new questions and opportunities emerge for forward-thinking business leaders.
How will these agentic systems change workplace dynamics? What new capabilities do they bring to organizations? How can businesses implement them effectively while navigating the technical and cultural challenges they present?
In this article, we'll explore the transformative potential of agentic AI co-workers, examining how they differ from traditional chatbots, their key capabilities, practical applications across industries, and strategies for successful implementation. Whether you're just beginning your AI journey or looking to advance existing initiatives, understanding this evolution is crucial for maintaining competitive advantage in an increasingly AI-augmented business landscape.
The Evolution from Reactive Tools to Proactive Partners
The journey from chatbots to agentic co-workers reflects AI's broader evolution from narrowly-focused tools to sophisticated systems capable of collaboration and independent action.
First-generation chatbots emerged primarily as customer service solutions—rule-based systems following predetermined scripts with minimal understanding of context or nuance. They were designed to handle simple, repetitive queries, reducing the burden on human agents but offering little beyond cost reduction and basic efficiency.
As natural language processing advanced, second-generation conversational AI developed greater linguistic capabilities and some contextual understanding. These systems could handle more complex interactions but remained fundamentally reactive—waiting for human prompts before taking action and constrained to specific domains.
The shift to agentic AI represents a quantum leap in this progression. Unlike their predecessors, agentic systems can:
- Initiate actions and conversations without being prompted
- Understand and adapt to changing contexts and priorities
- Learn continuously from interactions and outcomes
- Make decisions autonomously within defined parameters
- Collaborate with both humans and other AI systems
This evolution parallels how human working relationships develop—from giving specific instructions to new employees to eventually trusting experienced colleagues with independent projects and decision-making authority.
As one global financial institution's CTO recently observed: "We've moved from AI that simply responds to requests to systems that proactively identify issues, propose solutions, and implement them after appropriate review. It's fundamentally changing how we think about productivity and expertise in our organization."
Defining Agentic AI: What Makes These Systems Different
The term "agentic" derives from the concept of agency—the capacity to take action independently toward defined goals. This foundational characteristic distinguishes agentic co-workers from conventional AI systems in several crucial ways.
Traditional chatbots and automation tools follow linear processes with clearly defined inputs and outputs. They execute specific functions when triggered but lack the capacity to adapt their approach or take initiative beyond their programmed parameters.
Agentic AI systems, by contrast, operate with a degree of autonomy that allows them to:
Navigate Complexity and Ambiguity: Rather than following rigid scripts, agentic systems can handle uncertain or novel situations by applying reasoning capabilities and learned patterns from previous experiences.
Maintain Persistent Goals: Unlike transactional systems that complete discrete tasks and then stop, agentic co-workers maintain ongoing awareness of organizational objectives and continuously work toward them across multiple interactions and time periods.
Exhibit Self-Direction: Perhaps most distinctively, these systems can determine appropriate actions without explicit human direction. They identify needs, prioritize tasks, and initiate work based on their understanding of organizational goals and priorities.
Adapt to Feedback: Agentic systems continuously refine their approaches based on outcomes and human feedback, developing increasingly sophisticated models of what constitutes success in their domain.
This shift from purely reactive to proactive AI creates fundamentally different integration possibilities within organizations. While traditional AI tools augment specific processes, agentic systems can function as semi-autonomous team members that complement human capabilities across multiple domains simultaneously.
Consider the contrast between a customer service chatbot that responds to specific questions and an agentic customer success AI that proactively monitors customer health signals, identifies at-risk accounts, prepares personalized retention strategies, and initiates outreach at optimal times—all while continuously learning from results to improve future approaches.
Key Capabilities of AI Co-workers
Agentic AI co-workers bring a distinctive set of capabilities to organizations that extend well beyond traditional automation and analytics tools. Understanding these capabilities is essential for identifying the highest-value implementation opportunities.
Autonomous Learning and Adaptation
Unlike static systems that require regular reprogramming, agentic co-workers employ sophisticated machine learning techniques to improve continuously through experience. They analyze outcomes, identify patterns in successful and unsuccessful approaches, and refine their methods accordingly.
A manufacturing company implementing agentic quality control systems reported that predictive accuracy improved 34% over six months without manual intervention as the system learned from production data and outcomes.
Contextual Intelligence
Agentic systems maintain awareness of organizational context including business priorities, team structures, and situational factors. This contextual intelligence allows them to adjust their approach based on circumstances rather than applying one-size-fits-all solutions.
For example, an agentic project management assistant might prioritize different aspects of a project based on its current phase, stakeholder concerns, or changes in resource availability—much as an experienced human project manager would.
Multi-modal Communication
Advanced agentic systems can communicate through multiple channels and formats, selecting the most appropriate medium for different types of information and stakeholders. This might include generating visualizations for complex data, crafting written summaries of findings, or providing concise verbal updates.
Principled Operation
Sophisticated agentic systems operate according to defined principles and constraints that reflect organizational values and priorities. These guardrails ensure that autonomous actions align with business needs and ethical considerations.
A financial services firm implementing agentic compliance monitoring established clear parameters around risk tolerance, regulatory requirements, and escalation protocols, allowing their system to independently handle routine compliance checks while referring complex cases to human experts.
Coordination and Collaboration
Perhaps most significantly, agentic co-workers excel at coordination—working effectively with humans and other systems to accomplish shared objectives. This includes:
- Aligning activities with team priorities
- Providing appropriate transparency into their reasoning
- Recognizing when human intervention is needed
- Adapting to individual working styles
- Sharing relevant information proactively
These collaborative capabilities enable effective integration into existing teams and workflows, creating genuinely complementary human-AI partnerships rather than isolated automation islands.
Real-World Applications Across Industries
The transition from basic chatbots to agentic co-workers is creating transformative opportunities across sectors. Organizations at the forefront of this shift are discovering applications that go far beyond cost reduction to create new capabilities and competitive advantages.
Financial Services
Financial institutions are deploying agentic systems that combine regulatory expertise with continuous monitoring capabilities:
- Risk Management: AI co-workers that continuously analyze market conditions, portfolio performance, and emerging threats to provide proactive risk mitigation recommendations
- Client Advisory: Systems that blend financial planning expertise with personalized insights, proactively identifying opportunities and concerns specific to individual clients
- Regulatory Compliance: Agentic co-workers that monitor transactions, documentation, and regulatory changes to ensure continuous compliance while adapting to evolving requirements
A regional bank reported 78% faster regulatory reporting and a 42% reduction in compliance-related exceptions after implementing agentic compliance co-workers that could autonomously prepare regulatory documentation and identify potential issues before submission.
Healthcare and Life Sciences
Healthcare organizations are implementing agentic systems to enhance both clinical and operational functions:
- Clinical Decision Support: AI colleagues that integrate patient data, medical literature, and treatment protocols to suggest personalized care approaches
- Research Acceleration: Systems that autonomously analyze research literature, identify promising avenues for investigation, and even design experimental protocols
- Care Coordination: Agentic co-workers that proactively manage patient journeys across care settings, ensuring information transfer and appropriate follow-up
A pharmaceutical company reduced drug discovery timelines by 34% using agentic research assistants that could independently review scientific literature, propose molecular candidates, and design screening protocols without constant human direction.
Manufacturing and Supply Chain
Manufacturing operations benefit from agentic systems that bring continuous optimization and predictive capabilities:
- Predictive Maintenance: AI co-workers that monitor equipment performance, predict potential failures, and autonomously schedule maintenance during optimal production windows
- Supply Chain Resilience: Systems that continuously evaluate supplier performance, identify potential disruptions, and recommend diversification strategies before problems occur
- Quality Optimization: Agentic colleagues that analyze production data to identify subtle factors affecting product quality and recommend process adjustments
A global manufacturer implemented agentic supply chain co-workers that reduced disruption-related downtime by 67% by proactively identifying potential supplier issues and initiating contingency measures without waiting for problems to emerge.
Professional Services
Consulting, legal, and other professional services firms are leveraging agentic co-workers to augment knowledge work:
- Research and Analysis: Systems that independently gather information, identify patterns, and synthesize findings into actionable insights
- Document Creation: AI colleagues that draft contracts, reports, and presentations based on organizational templates and historical examples
- Knowledge Management: Agentic systems that continuously organize organizational knowledge and proactively share relevant information with team members
A global consulting firm deployed agentic research assistants that reduced time spent on preliminary research by 62%, allowing consultants to focus on higher-value analysis and client interaction.
Across these applications, the common theme is a shift from AI that performs isolated tasks to systems that function as true team members—taking initiative, adapting to circumstances, and collaborating effectively with human colleagues.
Implementation Challenges and Solutions
Transitioning from basic AI tools to agentic co-workers involves significant challenges across technical, organizational, and human dimensions. Organizations that have successfully navigated this evolution typically address several key areas:
Technical Infrastructure and Data Readiness
Challenge: Agentic systems require access to diverse data sources, integration across multiple systems, and robust computational resources.
Solution: Successful organizations typically begin with a focused data readiness assessment, identifying the specific information sources needed for initial use cases. Rather than attempting enterprise-wide data integration immediately, they create purpose-built data pipelines for specific AI applications while developing a longer-term data strategy.
A retail organization beginning their agentic AI journey focused first on integrating customer service, purchase history, and product data to support customer experience use cases before expanding to operational applications.
Skills and Capability Development
Challenge: Most organizations lack the specialized expertise needed to implement and manage agentic AI systems effectively.
Solution: Leading companies are addressing this gap through multi-faceted approaches:
- Creating hybrid teams that combine internal domain experts with external AI specialists
- Developing structured learning programs focused on AI collaboration skills
- Establishing centers of excellence to build institutional knowledge
- Participating in external AI workshops and masterclasses to accelerate capability development
As one healthcare CIO explained: "We recognized that neither technical skills nor domain expertise alone was sufficient. We needed to build bridges between these worlds through focused training and collaborative projects."
Governance and Control Frameworks
Challenge: Agentic systems require clear parameters around autonomy, decision rights, and oversight to operate effectively while maintaining appropriate controls.
Solution: Forward-thinking organizations are developing tiered governance models that define:
- Categories of decisions different AI systems can make independently
- Circumstances requiring human review or approval
- Monitoring and audit mechanisms for AI actions
- Clear accountability for outcomes of AI-initiated actions
These frameworks typically start with conservative autonomy boundaries that expand as systems demonstrate reliability and the organization builds confidence.
Change Management and Cultural Adaptation
Challenge: The introduction of agentic co-workers often triggers uncertainty and resistance from employees concerned about job displacement or changes to established ways of working.
Solution: Successful implementations emphasize augmentation over replacement, with clear communication about how human roles will evolve. Organizations that frame agentic AI as enabling people to focus on higher-value work typically see faster adoption and less resistance.
Effective approaches include:
- Involving end-users in system design and implementation
- Creating visible early wins that demonstrate value to affected teams
- Celebrating examples of effective human-AI collaboration
- Providing transition support for roles most affected by AI implementation
A financial services firm implementing agentic compliance systems established a "co-pilot" model where AI systems worked alongside existing compliance staff for six months before gradually assuming more independent responsibilities, creating time for human staff to transition to more strategic roles.
Ethical Considerations and Responsible Implementation
Challenge: Agentic systems raise important questions about transparency, fairness, and accountability that must be addressed proactively.
Solution: Leading organizations are establishing dedicated AI ethics committees and developing clear principles for responsible AI deployment. These typically include:
- Transparency requirements for AI decision-making
- Regular auditing for potential biases in AI operations
- Clear attribution of accountability for AI-initiated actions
- Ongoing monitoring of societal and ethical implications
Many organizations are finding that external consulting expertise can help navigate these complex implementation challenges while accelerating time-to-value and reducing risk.
The Human-AI Collaboration Model
As agentic co-workers become integrated into business operations, a new model of human-AI collaboration is emerging—one that differs significantly from traditional ways of working with technology. This model recognizes AI systems not merely as tools but as genuine collaborators with distinct capabilities and limitations.
Complementary Intelligence
The most effective human-AI partnerships leverage the complementary strengths of each party. While agentic systems excel at processing vast quantities of information, maintaining consistent performance, and operating continuously, humans bring creativity, ethical judgment, interpersonal skills, and contextual understanding that AI currently lacks.
A global telecommunications company restructured its customer experience teams around this principle, with agentic systems handling pattern recognition across millions of customer interactions while human agents focused on complex problem-solving and emotional connection.
Communication and Transparency
Effective collaboration requires transparent communication in both directions. Leading organizations are developing interaction models where:
- AI systems clearly explain their reasoning and confidence levels
- Humans provide specific feedback that AI systems can learn from
- Both parties establish shared vocabulary and reference points
- Communication adapts to context and urgency appropriately
A professional services firm developed a structured feedback protocol for their document analysis AI, allowing subject matter experts to efficiently communicate errors or improvements in a format that directly enhanced the system's learning.
Evolving Role Boundaries
As agentic systems mature, the boundaries between human and AI responsibilities naturally evolve. Organizations seeing the greatest success approach this as a dynamic partnership rather than a fixed division of labor.
Initially, AI co-workers typically handle well-defined, routine aspects of processes with clear human oversight. As trust and capability develop, they gradually assume more complex responsibilities and greater autonomy, while humans shift toward exception handling, relationship management, and strategic direction.
A manufacturing company began with agentic quality monitoring systems that simply flagged potential issues for human review. As performance data demonstrated reliability, these systems progressively gained authority to make routine adjustments independently, eventually managing entire quality parameters while humans focused on systemic improvements and innovation.
Psychological and Cultural Dimensions
The transition to working with AI colleagues involves psychological and cultural adaptations that organizations must address intentionally:
- Developing appropriate trust (neither over-reliance nor unwarranted skepticism)
- Creating comfort with human-AI collaboration as a normal working model
- Establishing new management practices for hybrid human-AI teams
- Evolving performance metrics to reflect collaborative outcomes
A financial services leader noted: "We found that the technical implementation was relatively straightforward compared to the cultural shift required. Building the right collaborative mindset was essential for realizing the technology's potential."
Organizations that thoughtfully design these collaboration models typically report significantly greater benefits than those that simply deploy advanced AI technology without considering the human side of the equation.
Measuring Business Impact and ROI
Quantifying the return on investment from agentic AI implementation requires a multidimensional approach that captures both immediate operational impacts and longer-term strategic benefits. Organizations at the forefront of this transition are developing comprehensive measurement frameworks that address several key areas:
Operational Efficiency and Productivity
The most immediately measurable benefits typically appear in operational metrics:
- Process Cycle Time: Reductions in end-to-end process duration, often 30-70% for knowledge-intensive processes
- Throughput per Employee: Increases in output per human team member when augmented by agentic co-workers
- Capacity Expansion: Ability to handle greater volume without proportional staffing increases
- Error Reduction: Decreased exception rates and rework requirements
A financial services firm implementing agentic compliance co-workers documented a 64% reduction in document review time, 42% fewer compliance exceptions, and the ability to handle a 30% increase in transaction volume without additional headcount.
Quality and Consistency Improvements
Beyond efficiency, agentic systems typically deliver significant quality enhancements:
- Consistency: More uniform application of best practices across operations
- Accuracy: Reduced error rates in information processing and analysis
- Completeness: More thorough consideration of relevant factors in decisions
- Compliance: Higher adherence to regulatory requirements and internal policies
A healthcare organization found that diagnostic recommendations from agentic clinical decision support systems demonstrated 28% greater protocol adherence and considered 3.4 times more relevant factors from patient histories compared to standard approaches.
Business Outcomes and Strategic Benefits
The full value of agentic co-workers extends to broader business metrics:
- Revenue Impact: Enhanced customer experience leading to improved retention and expansion
- Market Responsiveness: Faster adaptation to changing conditions and opportunities
- Innovation Acceleration: Increased capacity for new product development and optimization
- Risk Reduction: Early identification and mitigation of potential problems
A retail organization attributed a 23% increase in customer lifetime value to the personalized engagement capabilities of their agentic customer experience systems, which could proactively identify and address individual needs at scale.
ROI Timelines and Measurement Approaches
Organizations successfully measuring ROI typically apply staged evaluation frameworks that recognize the evolving nature of benefits:
- Initial Phase (0-6 months): Focus on operational metrics and direct cost impacts
- Intermediate Phase (6-18 months): Expand to quality improvements and initial business outcome measures
- Mature Phase (18+ months): Incorporate strategic benefits and competitive differentiation metrics
Importantly, these frameworks account for the continuous learning nature of agentic systems, recognizing that ROI typically accelerates over time as AI systems gain experience within the specific organizational context.
A manufacturing leader observed: "Unlike traditional automation projects where benefits plateau after implementation, our agentic systems continue to improve quarterly, creating compound returns on our initial investment."
Companies implementing robust measurement approaches typically find that agentic AI investments deliver positive returns within 6-12 months, with ROI increasing substantially in subsequent years as systems mature and use cases expand.
Future Outlook: The Next Evolution
While the shift from chatbots to agentic co-workers represents a significant advancement, it marks just the beginning of an ongoing evolution in how AI integrates into business operations. Forward-thinking executives are already preparing for several emerging developments that will further transform the landscape:
Collaborative AI Networks
The next frontier involves networks of specialized AI agents working together to accomplish complex objectives. Rather than single systems attempting to handle all aspects of a process, these networks will feature specialized agents for different functions—research, analysis, creativity, execution, quality control—coordinating their efforts much like human teams.
Early implementations in product development have demonstrated how research-focused agents can continuously gather market intelligence, collaborative with creative agents to generate concepts, and coordinate with evaluation agents to test ideas—all functioning as an integrated innovation team.
Advanced Multimodal Capabilities
Current agentic systems primarily process text, numbers, and limited visual information. Next-generation systems will feature sophisticated multimodal capabilities—understanding images, video, audio, physical spaces, and human emotions with much greater fidelity.
This will enable applications like manufacturing co-workers that can "see" production processes in real-time, customer experience agents that recognize emotional cues during interactions, and creative collaborators that can work with visual and auditory concepts alongside text.
Ambient Intelligence and Spatial Computing
Agentic AI is expanding beyond screens and devices into physical environments through ambient intelligence—systems that understand and respond to the context of physical spaces and human activities within them.
Health systems are pioneering applications where AI co-workers function as ambient clinical assistants, observing patient encounters, automatically documenting relevant information, suggesting potential diagnoses, and retrieving pertinent medical references without explicit commands.
Evolutionary Learning Systems
While current AI systems learn primarily through direct feedback, future agentic co-workers will employ evolutionary approaches that test variations of strategies to identify optimal approaches autonomously.
This capability will enable systems that continuously experiment with different methods for accomplishing goals—whether optimizing marketing approaches, refining operational processes, or developing new product features—learning from results to progressively improve performance without constant human guidance.
Trust and Relationship Dynamics
Perhaps most intriguingly, we're beginning to see the emergence of genuine trust relationships between humans and AI systems. As agentic co-workers demonstrate reliability, transparency, and alignment with human values over time, the nature of the collaboration evolves from transactional oversight to genuine partnership.
This evolution will increasingly influence organizational structures, management approaches, and even company culture as AI systems become integrated stakeholders in business operations.
For business leaders, these emerging trends underscore the importance of viewing agentic AI implementation not as a one-time project but as a capability development program that will evolve alongside the technology. Organizations that establish foundations for human-AI collaboration today will be best positioned to incorporate these advanced capabilities as they mature.
As one technology executive at a recent Business+AI Forum observed: "The organizations gaining the greatest advantage aren't necessarily those with the most advanced technology, but those that have developed the organizational capacity to integrate AI effectively into their operations and culture."
Preparing Your Organization for Agentic AI
The transition from chatbots to agentic co-workers represents a strategic opportunity that requires deliberate preparation across multiple dimensions. Organizations successfully navigating this evolution typically focus on several key areas:
Strategic Alignment and Vision
Rather than approaching AI implementation as a series of disconnected projects, leading organizations develop a coherent vision for how agentic systems will transform their operations and create competitive advantage.
This vision connects AI initiatives directly to strategic business objectives—whether enhancing customer experience, accelerating innovation, optimizing operations, or developing new business models. It serves as a north star for implementation decisions and helps maintain focus on high-value applications.
A global financial services firm began their journey by defining a clear "AI-augmented banking" vision that guided their transition from transactional chatbots to agentic systems that function as personalized financial advisors, risk management co-pilots, and regulatory compliance partners.
Organizational Readiness and Capability Building
Successful adoption requires developing new capabilities across technical, operational, and cultural dimensions:
- Technical Foundations: Data architecture, integration capabilities, and computing infrastructure that can support agentic systems
- Skills Development: Training programs that prepare both technical teams and end-users to work effectively with AI colleagues
- Process Redesign: Rethinking workflows to leverage the unique capabilities of human-AI collaboration rather than simply automating existing processes
- Governance Structures: Frameworks for managing AI systems throughout their lifecycle, from development through deployment and ongoing operation
Many organizations are finding that external partnerships accelerate this preparation process. Specialized workshops focused on AI implementation readiness and expert consulting can help bridge capability gaps while internal teams develop necessary skills.
Phased Implementation Approach
Rather than attempting wholesale transformation, successful organizations typically adopt phased approaches that balance ambition with pragmatism:
- Pilot Phase: Select narrowly defined use cases with clear success metrics to build experience and demonstrate value
- Expansion Phase: Apply lessons from initial implementations to broader applications while developing internal capabilities
- Transformation Phase: Reimagine core business processes and models based on the distinctive capabilities of agentic systems
This graduated approach allows organizations to learn and adapt while managing risk and building internal support through demonstrated successes.
Human-Centered Design and Change Management
Perhaps most critically, organizations that achieve the greatest impact maintain a relentless focus on the human side of the equation—designing AI implementations around human needs and capabilities rather than technology alone.
This includes:
- Involving end-users in the design process from the beginning
- Creating clear expectations about how roles will evolve alongside AI capabilities
- Celebrating and sharing examples of successful human-AI collaboration
- Providing support for employees transitioning to new ways of working
A healthcare system implementing agentic clinical decision support engaged physicians as design partners from the outset, ensuring that AI capabilities enhanced rather than disrupted their clinical workflows and decision processes.
Learning Community Participation
Given the rapidly evolving nature of agentic AI, organizations benefit significantly from participating in learning communities where they can share experiences, exchange best practices, and stay informed about emerging capabilities and approaches.
These communities—whether industry consortia, academic partnerships, or business forums—provide critical intelligence and learning opportunities that help organizations adapt their strategies as technologies and applications mature.
The path from chatbots to agentic co-workers represents one of the most significant opportunities for business transformation in a generation. Organizations that approach this transition thoughtfully, with attention to both technological and human dimensions, will be best positioned to capture the substantial benefits these systems can deliver.
For executives looking to accelerate their organization's journey, Business+AI's membership program provides access to implementation frameworks, expert guidance, and a community of peers navigating similar challenges—resources that can significantly reduce the learning curve and increase the probability of successful transformation.
The evolution from simple chatbots to sophisticated agentic co-workers represents a fundamental shift in how businesses leverage artificial intelligence. This transition goes far beyond incremental improvements in automation—it reimagines the relationship between humans and technology in the workplace, creating new possibilities for collaboration, innovation, and competitive advantage.
As we've explored throughout this article, agentic AI systems bring distinctive capabilities that enable them to function as true colleagues rather than mere tools. Their ability to operate autonomously, learn continuously, understand context, and collaborate effectively creates opportunities for transformation across virtually every business function and industry sector.
Yet realizing these benefits requires more than just implementing advanced technology. Organizations that successfully navigate this transition approach it as a multifaceted transformation that encompasses technical infrastructure, talent development, process redesign, governance frameworks, and cultural adaptation.
The organizations gaining the greatest advantage aren't viewing agentic AI as a collection of point solutions but as a strategic capability that can fundamentally enhance how they create value. They're developing clear visions for human-AI collaboration, building necessary foundations, and implementing thoughtful change management approaches that bring their people along on the journey.
As this field continues to evolve, staying connected with peers, experts, and emerging best practices becomes increasingly important. The organizations that combine technological implementation with organizational learning will be best positioned to capture the transformative potential of agentic co-workers.
Ready to transform how your organization leverages AI? Business+AI's membership program brings together executives, AI experts, and implementation specialists to help you navigate the shift from basic AI tools to sophisticated agentic co-workers. Join a community of forward-thinking leaders turning AI potential into tangible business results through hands-on workshops, masterclasses, and expert-led implementation support.