Developing Your First AI Strategy: A Simple Framework for Business Success
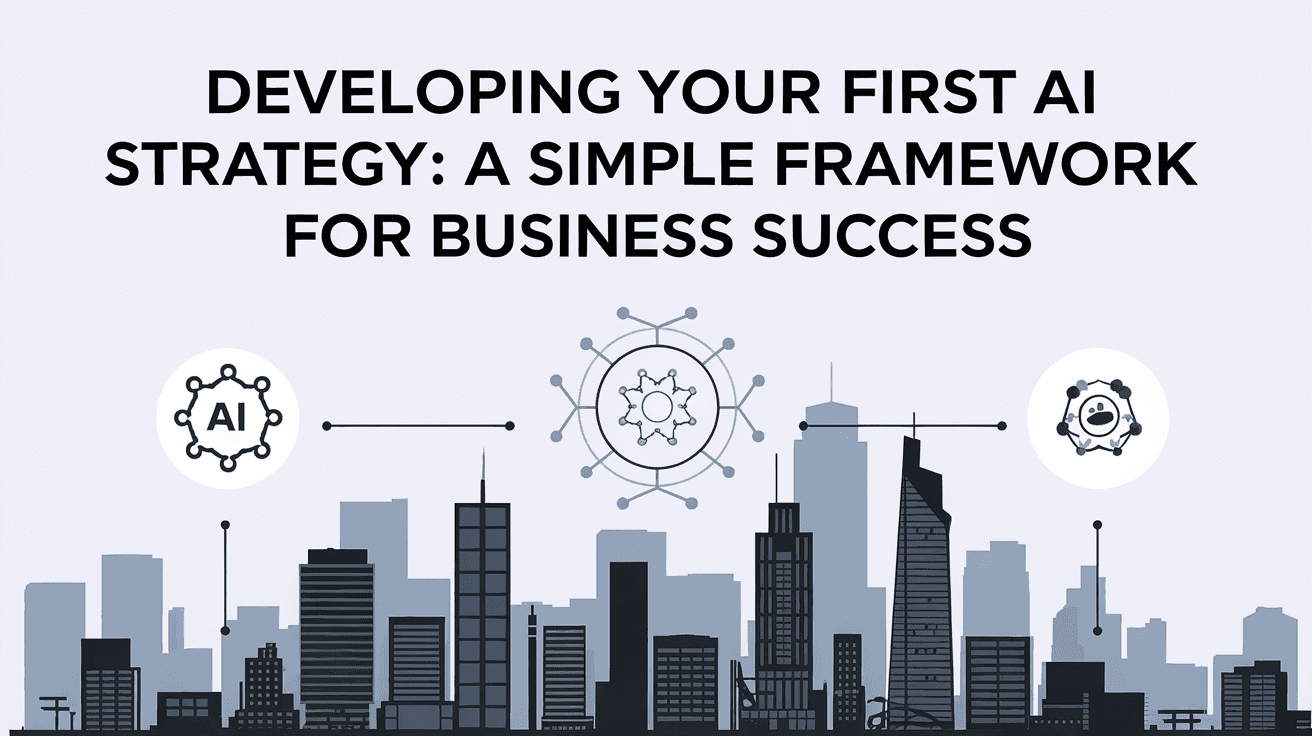
Table of Contents
- Why Every Business Needs an AI Strategy
- Understanding the AI Strategy Landscape
- The 5-Step AI Strategy Framework
- Implementation Challenges and Solutions
- Measuring Success: KPIs for Your AI Initiatives
- Next Steps: From Strategy to Execution
Developing Your First AI Strategy: A Simple Framework for Business Success
In today's rapidly evolving business landscape, artificial intelligence (AI) has transitioned from a futuristic concept to a critical business enabler. Despite this shift, many organizations struggle to move beyond theoretical discussions and pilot projects to implement AI solutions that deliver meaningful business value. According to McKinsey, only 26% of companies report significant bottom-line impact from AI initiatives, highlighting the gap between AI potential and realized business outcomes.
This disconnect often stems from a fundamental issue: the absence of a coherent, business-aligned AI strategy. Without a structured approach to AI adoption, organizations risk investing in disconnected technologies that fail to address core business challenges or generate sustainable competitive advantage.
In this comprehensive guide, we'll walk you through a straightforward framework for developing your first AI strategy—one that bridges the gap between technological possibilities and business realities. Whether you're a small enterprise taking your first steps into AI or a larger organization looking to formalize your approach, this framework will help you create a roadmap for AI adoption that delivers tangible results.
Why Every Business Needs an AI Strategy
The question is no longer whether businesses should adopt AI, but how they should do so strategically. A well-crafted AI strategy serves several critical functions that random AI experimentation simply cannot deliver:
First, it aligns AI initiatives with business objectives, ensuring that your technology investments directly support your organizational goals rather than existing as isolated technical achievements. Second, it prioritizes AI opportunities based on business value and feasibility, helping you focus limited resources on high-impact applications. Third, it coordinates efforts across departments, preventing the proliferation of incompatible AI solutions and duplicate investments.
Perhaps most importantly, a comprehensive AI strategy helps organizations navigate the unique challenges of AI adoption, from data governance and ethics concerns to talent acquisition and change management. Without this strategic foundation, businesses often fall into common traps: pursuing AI for its own sake, underestimating implementation complexity, or failing to scale successful pilots.
Understanding the AI Strategy Landscape
Before diving into framework development, it's essential to understand what makes AI strategy different from traditional technology planning. Unlike conventional IT systems with predetermined functions and outcomes, AI solutions evolve based on the data they process and the feedback they receive.
AI technologies fundamentally change how organizations operate by enabling new capabilities that weren't previously possible—from real-time decision-making at scale to uncovering hidden patterns in massive datasets. This transformative potential requires rethinking not just technology infrastructure, but also business processes, organizational structures, and talent requirements.
Common pitfalls in early AI adoption include:
- Technology-first thinking: Focusing on AI capabilities rather than business problems
- Disconnected pilots: Launching isolated proof-of-concepts without a plan for integration or scaling
- Underestimating data requirements: Failing to address data quality, accessibility, and governance issues
- Neglecting organizational readiness: Not preparing the organization for the changes AI will bring
- Unrealistic expectations: Overestimating short-term impacts while underestimating long-term transformation
A robust AI strategy anticipates these challenges and creates frameworks to address them systematically.
The 5-Step AI Strategy Framework
Developing an effective AI strategy doesn't require an advanced degree in data science or months of planning. Our simplified framework breaks the process into five manageable steps that any organization can implement.
Step 1: Define Your Business Objectives
All successful AI strategies begin with clearly articulated business goals. Start by identifying your organization's strategic priorities and pain points that AI could potentially address. Ask questions like:
- What are our most significant business challenges or opportunities?
- Which processes consume disproportionate resources?
- Where do we need to make faster or more accurate decisions?
- What information or insights would transform our business if available?
The key is to focus on business outcomes rather than AI capabilities. Instead of asking, "How can we use natural language processing?" ask, "How can we improve our customer response time and quality?" This business-first approach ensures that your AI initiatives directly contribute to organizational objectives.
For example, a financial services company might identify several business objectives: reducing fraud, improving customer service response times, and enhancing investment decision-making. Each of these objectives could potentially be supported by AI, but they require different approaches and yield different types of business value.
Step 2: Assess Your AI Readiness
Before committing resources to AI initiatives, conduct an honest assessment of your organization's readiness across four critical dimensions:
- Data readiness: Evaluate the quality, accessibility, and governance of your data. AI systems are only as good as the data they learn from.
- Technical infrastructure: Assess whether your current systems can support AI workloads or need upgrades.
- Talent and skills: Inventory existing AI capabilities and identify gaps that need filling through hiring, training, or partnerships.
- Organizational culture: Determine your organization's appetite for change and capacity to integrate AI into existing workflows.
This assessment helps identify prerequisites that must be addressed before AI implementation and informs your approach to capability building. At Business+AI workshops, we use a structured readiness assessment tool that helps organizations identify their strengths and gaps across these dimensions.
Step 3: Identify High-Value AI Opportunities
With business objectives defined and organizational readiness assessed, the next step is identifying specific AI use cases with the potential to deliver significant value. Evaluate potential opportunities using a structured framework that considers:
- Business impact: How significantly will this application affect key business metrics?
- Technical feasibility: How challenging will implementation be given current technology and your organization's capabilities?
- Data availability: Do you have access to the necessary data, or can you reasonably acquire it?
- Time to value: How quickly can you expect to see results?
Create a prioritized list of opportunities based on these factors, focusing initially on projects that combine high business impact with lower implementation complexity and shorter time to value—often called "quick wins."
For instance, our Business+AI consulting team recently helped a manufacturing client identify predictive maintenance as their highest-priority AI use case. It offered substantial cost savings through reduced downtime, was technically feasible given their existing sensor infrastructure, and could demonstrate value within months rather than years.
Step 4: Build Your AI Roadmap
With prioritized opportunities in hand, develop a phased implementation roadmap that sequences initiatives logically and builds capabilities over time. Your roadmap should include:
- Short-term projects (3-6 months): Quick wins that deliver value while building organizational confidence and momentum
- Medium-term initiatives (6-18 months): More complex projects that may require additional capability building
- Long-term vision (18+ months): Transformative opportunities that might require significant organizational changes
Each phase should build upon previous successes, developing capabilities and generating insights that enable subsequent initiatives. Include specific milestones, resource requirements, and success metrics for each project.
Your roadmap should also account for foundational elements that support multiple AI initiatives, such as data infrastructure improvements, governance frameworks, and talent development programs.
Step 5: Establish Governance and Ethics Guidelines
The final component of your AI strategy framework should address how AI will be governed within your organization. This includes establishing policies and procedures for:
- Data governance: How data will be collected, stored, secured, and accessed for AI applications
- Ethical guidelines: Principles for responsible AI development and deployment
- Decision rights: Who has authority to approve AI initiatives and allocate resources
- Success measurement: How you'll evaluate and report on AI investments
Reliable governance structures become increasingly important as AI applications scale and become more embedded in critical business processes. Organizations that establish these guidelines early avoid many of the risks and challenges that come with more mature AI deployments.
During our Business+AI masterclass sessions, we help executives develop customized governance frameworks that balance innovation with appropriate oversight.
Implementation Challenges and Solutions
Even the best-designed AI strategies encounter obstacles during implementation. Anticipating common challenges and preparing to address them increases your likelihood of success.
Handling Data Quality Issues
Data quality problems frequently emerge as AI projects move from concept to implementation. These issues can range from missing values and inconsistent formats to biased samples and outdated information.
Address data challenges by starting with a thorough data audit before launching AI initiatives. Implement data quality improvement processes, establish clear data ownership, and invest in data engineering capabilities. Consider starting with smaller datasets you can clean and control while building more comprehensive data management capabilities.
Building Internal Capabilities
The AI talent shortage presents significant challenges for organizations developing their first AI strategy. Rather than competing directly for scarce data scientists, consider a multi-faceted approach:
- Upskill existing employees with domain expertise
- Partner with external specialists for specialized technical needs
- Leverage AI platforms and tools that require less specialized expertise
- Build a center of excellence that can share knowledge across the organization
The most successful organizations combine internal capability building with strategic external partnerships, gradually increasing their self-sufficiency as they mature.
Managing Change and Adoption
Technological implementation is only half the challenge; ensuring organizational adoption is equally critical. Resistance often stems from concerns about job displacement, changing roles, or unfamiliar workflows.
Address these challenges through comprehensive change management that includes:
- Clear communication about the purpose and benefits of AI initiatives
- Involvement of end-users in design and implementation
- Adequate training and support during transitions
- Visible executive sponsorship and leadership
Many AI initiatives fail not because of technological shortcomings but because of insufficient attention to the human and organizational aspects of change.
Measuring Success: KPIs for Your AI Initiatives
Effective AI strategies include clear metrics for measuring success. These should connect directly to the business objectives identified in Step 1 and might include:
- Efficiency metrics: Cost reduction, time savings, productivity improvements
- Quality metrics: Error reduction, accuracy improvements, consistency gains
- Revenue metrics: Sales increases, customer retention, market share growth
- Strategic metrics: New product development, market expansion, competitive differentiation
Implement both leading indicators (early signs of success) and lagging measures (final outcomes) to track progress. For example, a customer service AI might track immediate metrics like response time and resolution rate, alongside longer-term measures like customer satisfaction and retention.
Regular review of these metrics allows for course correction and helps build the case for further AI investments. At our annual Business+AI Forum, organizations share benchmarking data and best practices for measuring AI's business impact across industries.
Next Steps: From Strategy to Execution
With your AI strategy framework in place, the next challenge is translating plans into action. Begin by selecting one or two high-priority initiatives from your roadmap and establishing dedicated teams to drive them forward.
Create detailed implementation plans for these initial projects, including specific timelines, resource allocations, and success criteria. Consider using an agile approach that allows for rapid prototyping, testing, and refinement based on real-world feedback.
As you move forward, continue building the foundational capabilities identified in your readiness assessment. This might include enhancing data infrastructure, establishing AI governance committees, or developing training programs for employees.
Remember that successful AI adoption is a journey rather than a destination. Your strategy should evolve as you learn from early implementations and as AI technologies and applications continue to advance.
Conclusion
Developing your first AI strategy doesn't have to be overwhelming. By following this simple framework—defining business objectives, assessing readiness, identifying opportunities, building a roadmap, and establishing governance—you can create a practical approach to AI that delivers tangible business value.
The most successful AI strategies maintain a relentless focus on business outcomes while building the foundational capabilities needed for sustainable AI adoption. They balance short-term wins with long-term transformation, and they recognize that people and processes are as important as technology in realizing AI's potential.
Remember that your initial AI strategy is a starting point, not the final word. As you implement your first AI initiatives, you'll gain insights that help refine and evolve your approach. The key is getting started with a structured framework that connects AI possibilities to your specific business needs.
Ready to accelerate your AI journey? Join the Business+AI membership program to access expert guidance, peer networking, and exclusive resources that will help you implement your AI strategy with confidence. From workshops and masterclasses to personalized consulting, we provide the support you need to transform AI potential into business results.